EEG (electroencephalogram) signal unsupervised feature learning method based on convolutional network and self encoding
A convolutional network and feature learning technology, applied in the field of convolutional networks, can solve problems such as difficulty in feature design and selection, new patients are unknown, etc.
- Summary
- Abstract
- Description
- Claims
- Application Information
AI Technical Summary
Problems solved by technology
Method used
Image
Examples
Embodiment Construction
[0050] The following embodiments will further illustrate the present invention in conjunction with the accompanying drawings
[0051] Embodiments of the present invention include the following steps:
[0052] 1) The value of each dimension of the required EEG data sample is controlled between (0,1). Through 0-1 standardization, the sample data is linearly transformed, and the data is mapped to [0,1]. The conversion function is as follows:
[0053]
[0054] Where d is the value of one dimension of the input sample x, max(data) is the maximum value of each dimension in all samples, and min(data) is the minimum value of each dimension in all samples.
[0055] 2) Construct AE-CDNN model based on deep convolutional network and self-encoder. The framework structure of the deep convolutional network model (AE-CDNN) is shown in figure 1 . The model is mainly divided into two stages. The encoding stage operation includes sample input, convolutional layer, pooling layer (downsamp...
PUM
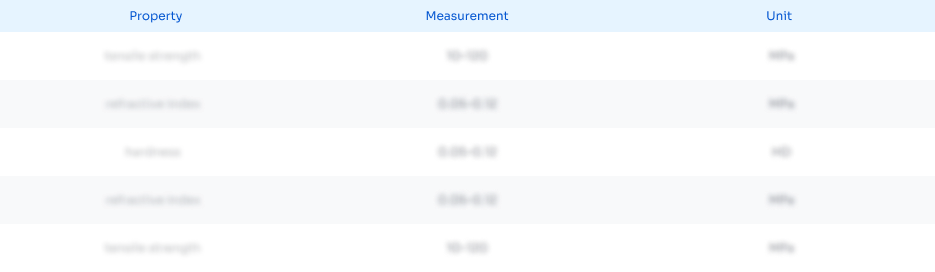
Abstract
Description
Claims
Application Information
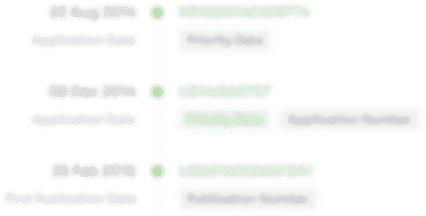
- R&D Engineer
- R&D Manager
- IP Professional
- Industry Leading Data Capabilities
- Powerful AI technology
- Patent DNA Extraction
Browse by: Latest US Patents, China's latest patents, Technical Efficacy Thesaurus, Application Domain, Technology Topic, Popular Technical Reports.
© 2024 PatSnap. All rights reserved.Legal|Privacy policy|Modern Slavery Act Transparency Statement|Sitemap|About US| Contact US: help@patsnap.com