Fault prediction method based on migrated convolutional neural network
A neural network and fault prediction technology, applied in the field of fault prediction based on migration convolutional neural network, can solve the problem of low fault prediction accuracy, achieve the effects of fast prediction speed, prevention of excessive cooperation, and simple conversion process
- Summary
- Abstract
- Description
- Claims
- Application Information
AI Technical Summary
Problems solved by technology
Method used
Image
Examples
Embodiment Construction
[0028] In order to make the object, technical solution and advantages of the present invention clearer, the present invention will be further described in detail below in conjunction with the accompanying drawings and embodiments. It should be understood that the specific embodiments described here are only used to explain the present invention, not to limit the present invention. In addition, the technical features involved in the various embodiments of the present invention described below can be combined with each other as long as they do not constitute a conflict with each other.
[0029] figure 1 It is a flow chart of the migration convolutional neural network fault prediction method constructed according to the preferred embodiment of the present invention, such as figure 1 As shown, the fault prediction method based on the migration convolutional neural network is characterized in that the method includes the following steps:
[0030] (a) Number the fault types of the...
PUM
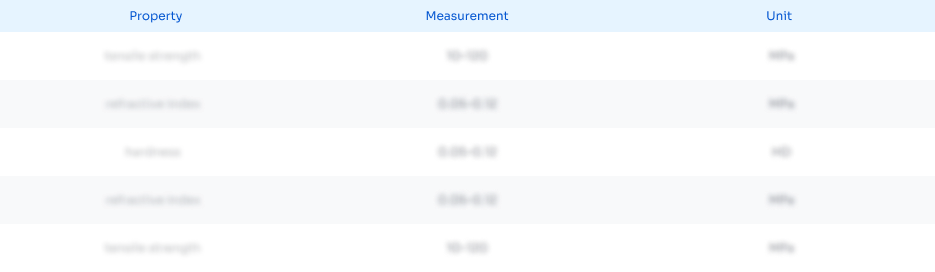
Abstract
Description
Claims
Application Information
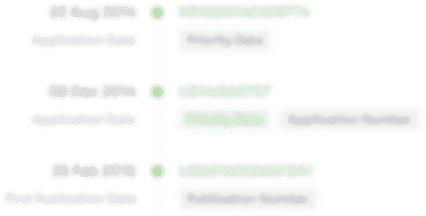
- R&D Engineer
- R&D Manager
- IP Professional
- Industry Leading Data Capabilities
- Powerful AI technology
- Patent DNA Extraction
Browse by: Latest US Patents, China's latest patents, Technical Efficacy Thesaurus, Application Domain, Technology Topic, Popular Technical Reports.
© 2024 PatSnap. All rights reserved.Legal|Privacy policy|Modern Slavery Act Transparency Statement|Sitemap|About US| Contact US: help@patsnap.com