Establishing method of rolling bearing intelligent diagnosis model based on convolutional neural network
A convolutional neural network and rolling bearing technology, which is applied in the field of building an intelligent diagnosis model for rolling bearings, to achieve the effect of improving the feature extraction ability and overcoming the mastery of a large number of signal processing technologies
- Summary
- Abstract
- Description
- Claims
- Application Information
AI Technical Summary
Problems solved by technology
Method used
Image
Examples
Embodiment Construction
[0033] Aiming at the problems that the modern equipment diagnosis technology using signal processing technology is difficult to analyze the simultaneous occurrence of multiple faults and the possible interrelationship and influence between various faults, and the time-consuming and laborious manual analysis of large data, the present invention proposes a A fault diagnosis method based on convolutional neural network, by mapping one-dimensional vibration data to generate a two-dimensional image, and then using convolutional neural network to identify and classify bearing faults to determine the severity, fault location and fault type of bearing faults ; Not only can avoid manual analysis of various complex big data collected, saving time and effort, but also can solve the problem of multiple faults occurring at the same time and the possible existence of various faults in modern equipment diagnosis technology using signal processing technology. It is difficult to analyze clearly...
PUM
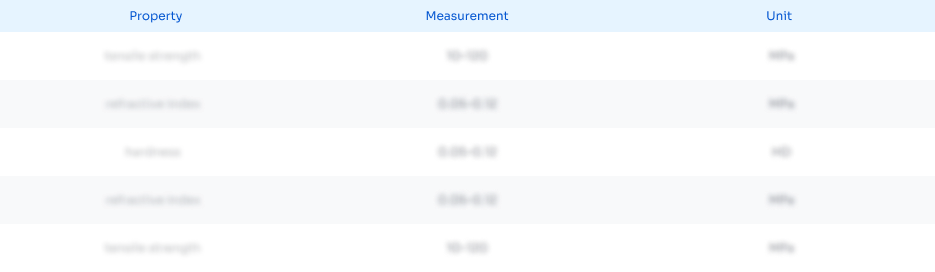
Abstract
Description
Claims
Application Information
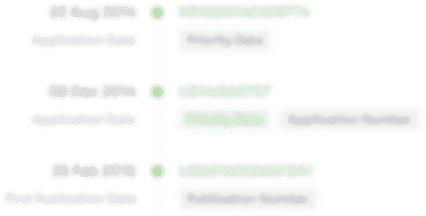
- R&D Engineer
- R&D Manager
- IP Professional
- Industry Leading Data Capabilities
- Powerful AI technology
- Patent DNA Extraction
Browse by: Latest US Patents, China's latest patents, Technical Efficacy Thesaurus, Application Domain, Technology Topic.
© 2024 PatSnap. All rights reserved.Legal|Privacy policy|Modern Slavery Act Transparency Statement|Sitemap