Hyperspectral image classification method based on convolutional network and cyclic neural network
A cyclic neural network, hyperspectral image technology, applied in character and pattern recognition, instrument, scene recognition and other directions, can solve the problems of insufficient and incomplete features, slow classification speed, low classification accuracy, etc., to reduce the amount of sample data, The effect of improving the classification speed and improving the classification accuracy
- Summary
- Abstract
- Description
- Claims
- Application Information
AI Technical Summary
Problems solved by technology
Method used
Image
Examples
Embodiment Construction
[0048] Below in conjunction with accompanying drawing, the present invention will be described in further detail
[0049] Refer to attached figure 1 , to further describe in detail the specific steps of the present invention.
[0050] Step 1. Construct a three-dimensional convolutional neural network.
[0051] Build a 7-layer three-dimensional convolutional neural network, and its structure is as follows: input layer→1st convolutional layer→1st pooling layer→2nd convolutional layer→2nd pooling layer→1st Fully connected layer → second fully connected layer → classification layer.
[0052] Set the parameters of each layer of the three-dimensional convolutional neural network as follows:
[0053] Set the total number of input layer feature maps to 3.
[0054] Set the total number of feature maps of the first convolutional layer to 32, and the size of the convolution kernel to 5×5×5.
[0055] Set the downsampling filter size of the first pooling layer to 2×2×2.
[0056] Set ...
PUM
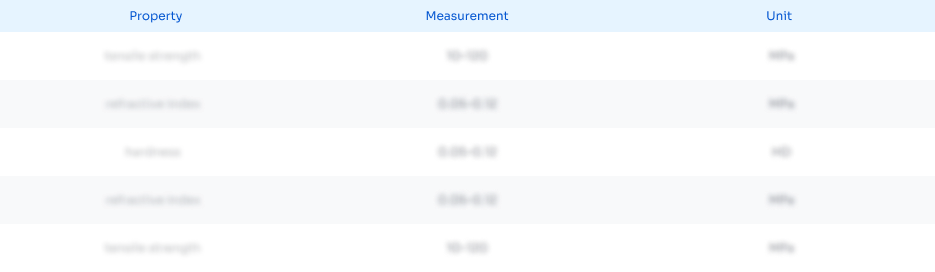
Abstract
Description
Claims
Application Information
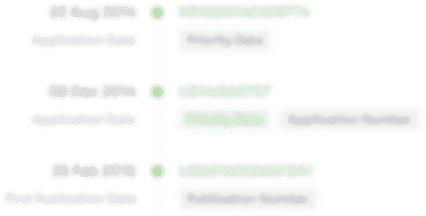
- R&D Engineer
- R&D Manager
- IP Professional
- Industry Leading Data Capabilities
- Powerful AI technology
- Patent DNA Extraction
Browse by: Latest US Patents, China's latest patents, Technical Efficacy Thesaurus, Application Domain, Technology Topic.
© 2024 PatSnap. All rights reserved.Legal|Privacy policy|Modern Slavery Act Transparency Statement|Sitemap