A Chord Invariant Forecasting Method for Short-term Bus Passenger Flow
A technology of passenger flow and passenger flow, which is applied in the field of computer and physics, and in the field of intelligent transportation, can solve problems such as difficult analysis, lack of data, and difficult passenger flow prediction at bus stations. high precision effect
- Summary
- Abstract
- Description
- Claims
- Application Information
AI Technical Summary
Problems solved by technology
Method used
Image
Examples
Embodiment Construction
[0042] The specific implementation manner of the present invention will be further described below in conjunction with the accompanying drawings and actual data.
[0043] This example uses the passenger flow survey and operation data of a bus line in a mega city in my country as an example. The following is the statistical table of the number of passengers boarding the bus on a typical weekday.
[0044]
[0045]
[0046] (1) The first 16 data in the table are extracted as the training set, and the genetic algorithm is used to train l s ,Q,η 1 , η 2 These four parameter values, the genetic algorithm flow chart attached figure 1 . Perform the following steps in conjunction with the flow chart:
[0047] 1) Set the SI-PFPM parameter set and parameter range, randomly generate a set of SI-PFPM parameters, encode the SI-PFPM parameters with binary coding, and determine the initial population size, mutation rate, and crossover rate.
[0048] 2) Determine the fitness functi...
PUM
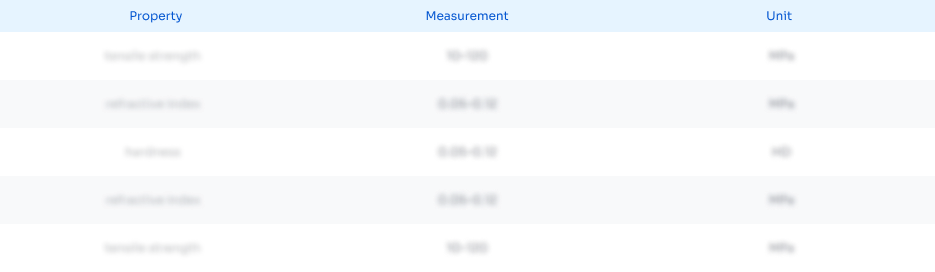
Abstract
Description
Claims
Application Information
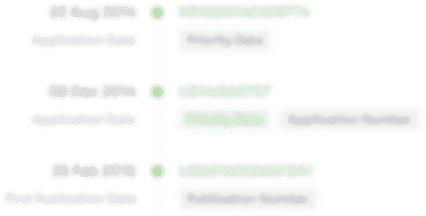
- Generate Ideas
- Intellectual Property
- Life Sciences
- Materials
- Tech Scout
- Unparalleled Data Quality
- Higher Quality Content
- 60% Fewer Hallucinations
Browse by: Latest US Patents, China's latest patents, Technical Efficacy Thesaurus, Application Domain, Technology Topic, Popular Technical Reports.
© 2025 PatSnap. All rights reserved.Legal|Privacy policy|Modern Slavery Act Transparency Statement|Sitemap|About US| Contact US: help@patsnap.com