Method for optimizing injection molding process based on RBF (Radial Basis Function) neural network
A neural network and process optimization technology, applied in the field of injection molding process, can solve problems such as difficult to eliminate, difficult to identify molding process parameters, and delayed mold production period.
- Summary
- Abstract
- Description
- Claims
- Application Information
AI Technical Summary
Problems solved by technology
Method used
Image
Examples
Embodiment Construction
[0041] see figure 1 As shown, the injection molding process optimization method based on RBF neural network of an embodiment provided by the invention comprises the following steps:
[0042] S100, establishing a CAE analysis model of the injection molding product and first injection molding parameters;
[0043] S200, performing at least one injection parameter optimization on the first injection parameters to obtain the first optimized injection parameters;
[0044] S300, performing an orthogonal test design on the first optimized injection molding parameters, and obtaining orthogonal test data;
[0045] S400, utilizing part or all of the orthogonal test data to train the RBF neural network to obtain the trained RBF neural network;
[0046] S500, using the trained RBF neural network to obtain the final optimized injection molding parameters.
[0047] The above-mentioned injection molding process optimization method based on RBF neural network, after the preliminary optimiza...
PUM
Property | Measurement | Unit |
---|---|---|
thickness | aaaaa | aaaaa |
Abstract
Description
Claims
Application Information
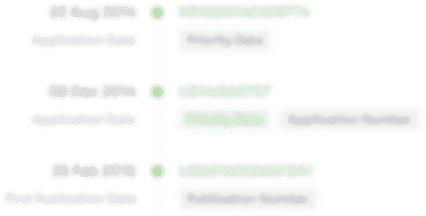
- R&D Engineer
- R&D Manager
- IP Professional
- Industry Leading Data Capabilities
- Powerful AI technology
- Patent DNA Extraction
Browse by: Latest US Patents, China's latest patents, Technical Efficacy Thesaurus, Application Domain, Technology Topic, Popular Technical Reports.
© 2024 PatSnap. All rights reserved.Legal|Privacy policy|Modern Slavery Act Transparency Statement|Sitemap|About US| Contact US: help@patsnap.com