Full convolution network based remote-sensing image land cover classifying method
A fully convolutional network and remote sensing image technology, applied in the field of remote sensing image surface coverage classification, can solve problems such as low computing efficiency and large storage overhead, and achieve the effect of improving performance, strong practicability, and broad application prospects.
- Summary
- Abstract
- Description
- Claims
- Application Information
AI Technical Summary
Problems solved by technology
Method used
Image
Examples
Embodiment Construction
[0033] The present invention will be further described in detail below in conjunction with the accompanying drawings and specific embodiments.
[0034] The present invention provides a remote sensing image land cover classification method based on a fully convolutional network, such as figure 1 shown, including the following steps:
[0035] Step S1: Carry out data enhancement on the data set with a limited amount of data, and generate a training set whose data amount and quality meet the training requirements.
[0036] The remote sensing image data set used for training is generally obtained by visual interpretation and manual drawing, which requires a lot of manpower, so it is necessary to use a certain data enhancement method for enhancement. First, the remote sensing images in the data set are stretched and scaled at different scales to increase the diversity of the data; then, the remote sensing images of a single large image in the data set are divided into blocks of the...
PUM
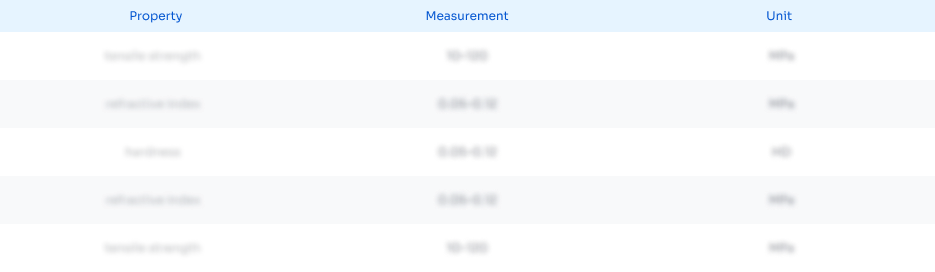
Abstract
Description
Claims
Application Information
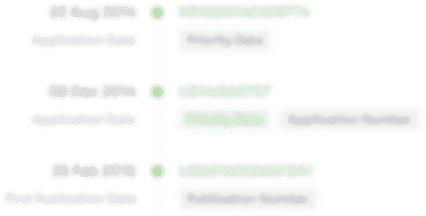
- R&D Engineer
- R&D Manager
- IP Professional
- Industry Leading Data Capabilities
- Powerful AI technology
- Patent DNA Extraction
Browse by: Latest US Patents, China's latest patents, Technical Efficacy Thesaurus, Application Domain, Technology Topic.
© 2024 PatSnap. All rights reserved.Legal|Privacy policy|Modern Slavery Act Transparency Statement|Sitemap