Image super-resolution reconstruction method
A super-resolution reconstruction and image technology, applied in the field of image processing, can solve problems such as inability to apply multiple magnifications, poor reconstruction effect, blurred edge information of the generated image, etc., to enhance the reconstruction effect, improve the reconstruction effect, and improve the convergence speed. Effect
- Summary
- Abstract
- Description
- Claims
- Application Information
AI Technical Summary
Problems solved by technology
Method used
Image
Examples
Embodiment 1
[0056] A specific embodiment of the present invention discloses a method for image super-resolution reconstruction, comprising the following steps:
[0057] S1. Construct a convolutional neural network for training and learning.
[0058] The convolutional neural network sequentially includes an LR feature extraction layer, a nonlinear mapping layer, and an HR reconstruction layer from top to bottom. Specifically, in the LR feature extraction layer, the gradient feature extraction is performed on the input LR image to obtain the LR feature map; in the nonlinear mapping layer, multiple nonlinear mappings are performed on the LR feature map to obtain the HR feature map; in the HR reconstruction layer, Perform image reconstruction on the HR feature map to obtain a HR reconstruction image.
[0059] S 2. Use the convolutional neural network to train the training LR image and the training HR image set in pairs in the input training library, and carry out training and learning of at ...
Embodiment 2
[0069] In another embodiment based on the above method, the convolutional neural network further includes an HR feature extraction layer, a loss function layer, a logic judgment module, and an HR gradient prior extraction layer. Among them, the HR feature extraction layer, the loss function layer, and the logical judgment module are set after the HR reconstruction layer in sequence, and the HR gradient prior extraction layer is set before the loss function layer, and are set side by side with the HR feature extraction layer.
[0070] The HR feature extraction layer performs gradient feature extraction on the HR reconstruction image output by the HR reconstruction layer to obtain the HR gradient feature map.
[0071] The HR gradient prior extraction layer extracts the gradient prior information from the training HR images in the training database (the resolution is the same as that of the HR reconstruction image, which is only used in the training process), and obtains the HR gr...
Embodiment 3
[0110] Such as image 3 As shown, in another embodiment based on the above method, the convolutional neural network under the scale of ×2 times, ×3 times, and ×4 times respectively shares the nonlinear mapping layer, by sharing the weight of the nonlinear mapping layer and For the receptive field, the same set of filters can be used for each path, and through information transfer at multiple scales, regularization guidance can be provided to each other, which greatly simplifies the complexity of the convolutional neural network and reduces the number of parameters.
[0111] Preferably, the nonlinear mapping layer includes three convolutional layers, for example, the above three convolutional layers are respectively marked as convolutional layers 21 , 22 , and 23 . The other layers are also marked sequentially, and will not be described here one by one.
[0112] In the LR feature extraction layer (convolutional layers 11, 12, 13), the output is expressed as:
[0113]
[01...
PUM
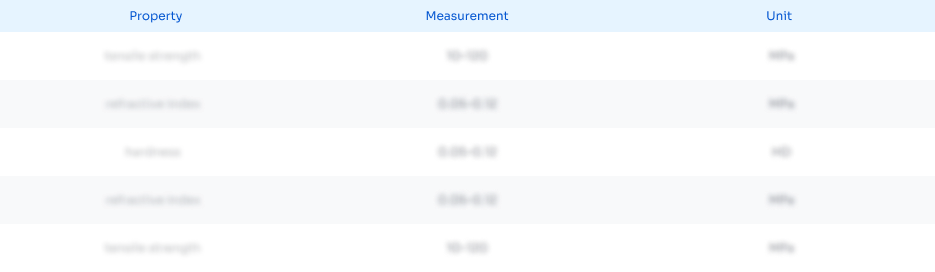
Abstract
Description
Claims
Application Information
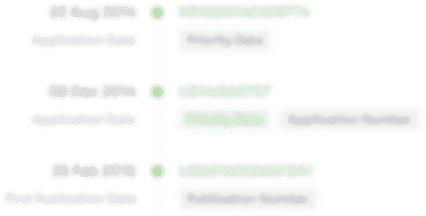
- R&D Engineer
- R&D Manager
- IP Professional
- Industry Leading Data Capabilities
- Powerful AI technology
- Patent DNA Extraction
Browse by: Latest US Patents, China's latest patents, Technical Efficacy Thesaurus, Application Domain, Technology Topic, Popular Technical Reports.
© 2024 PatSnap. All rights reserved.Legal|Privacy policy|Modern Slavery Act Transparency Statement|Sitemap|About US| Contact US: help@patsnap.com