Deep learning-based diabetic retina image classification method and system
A diabetic retina and deep learning technology, applied in neural learning methods, image analysis, medical images, etc., can solve the problems of medical images lagging behind natural images, difficult to obtain medical image data, and difficult to improve detection efficiency, so as to reduce the difficulty of development , strong applicability, and the effect of assisting clinical screening
- Summary
- Abstract
- Description
- Claims
- Application Information
AI Technical Summary
Problems solved by technology
Method used
Image
Examples
Embodiment 1
[0043] Such as figure 1 As shown, the present embodiment relates to a deep learning-based diabetic retinal image classification method, including:
[0044] Obtain the fundus image to be recognized;
[0045] The same fundus image to be recognized was imported into the microvascular tumor lesion recognition model, the hemorrhage lesion recognition model and the exudative lesion recognition model for recognition; the lesion feature information was extracted according to the recognition results, and then the extracted lesion was classified by the trained support vector machine classifier. Classify the feature information to obtain the lesion grade classification result corresponding to the fundus image;
[0046] The microangioma lesion recognition model is obtained by extracting the candidate area of microangioma lesion in the fundus image, marking the microangioma lesion area and the non-microangioma lesion area, and then inputting the CNN model for training;
[0047] The hem...
PUM
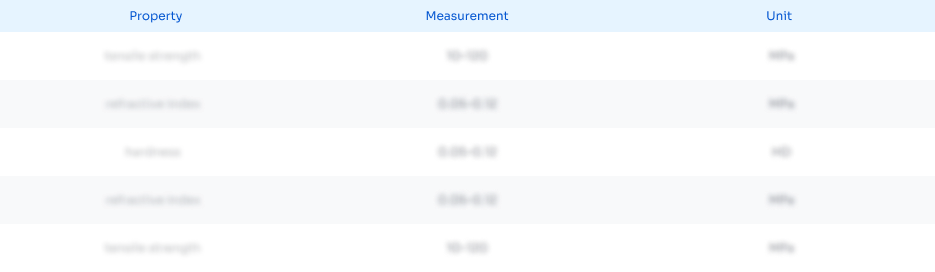
Abstract
Description
Claims
Application Information
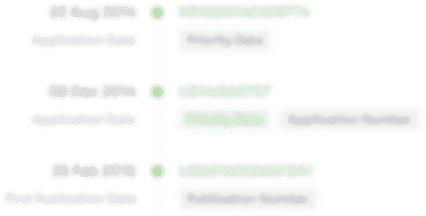
- R&D Engineer
- R&D Manager
- IP Professional
- Industry Leading Data Capabilities
- Powerful AI technology
- Patent DNA Extraction
Browse by: Latest US Patents, China's latest patents, Technical Efficacy Thesaurus, Application Domain, Technology Topic, Popular Technical Reports.
© 2024 PatSnap. All rights reserved.Legal|Privacy policy|Modern Slavery Act Transparency Statement|Sitemap|About US| Contact US: help@patsnap.com