An object detection method based on cross-modal and multi-scale feature fusion
A multi-scale feature and object detection technology, applied in the field of image recognition, can solve the problems of speed limitation, lack of inclusion, inability to directly obtain general feature expression of depth information, etc., to achieve real-time detection speed and improve detection performance.
- Summary
- Abstract
- Description
- Claims
- Application Information
AI Technical Summary
Problems solved by technology
Method used
Image
Examples
Embodiment Construction
[0028] The technical solution of the present invention will be described in further detail below in conjunction with the accompanying drawings and embodiments, and the following embodiments do not constitute a limitation of the present invention.
[0029] The general idea of the present invention is that, without relying on a large number of labeled depth image data sets, it is possible to fuse depth image and RGB image features across modalities, real-time, efficient, and accurately complete object recognition, positioning and detection. Train to obtain a fusion model that can accept cross-modal RGB and depth image input, and obtain the location and category information of multiple objects in real time. This solution needs to complete cross-modal feature transfer: initialize the depth map information network from the RGB model parameters and train the depth map model; and then initialize the feature extraction of the fusion network proposed by the present invention based on ...
PUM
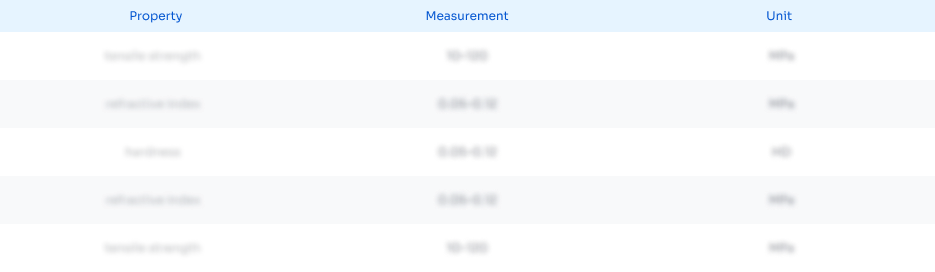
Abstract
Description
Claims
Application Information
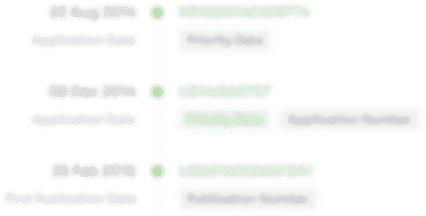
- R&D
- Intellectual Property
- Life Sciences
- Materials
- Tech Scout
- Unparalleled Data Quality
- Higher Quality Content
- 60% Fewer Hallucinations
Browse by: Latest US Patents, China's latest patents, Technical Efficacy Thesaurus, Application Domain, Technology Topic, Popular Technical Reports.
© 2025 PatSnap. All rights reserved.Legal|Privacy policy|Modern Slavery Act Transparency Statement|Sitemap|About US| Contact US: help@patsnap.com