Potential target area detection method based on convolutional neural network and conditional random field
A convolutional neural network and conditional random field technology, applied in the fields of deep learning and computer vision, can solve problems such as light changes, object color changes, noisy background interference, and insufficient robustness, so as to overcome the interference of color and background noise, The effect of reducing the number, improving positioning accuracy and robustness
- Summary
- Abstract
- Description
- Claims
- Application Information
AI Technical Summary
Problems solved by technology
Method used
Image
Examples
Embodiment
[0028] Such as figure 1 As shown, the potential target area detection method of the structured network based on convolutional neural network and conditional random field fusion of an example mode of the present invention comprises the following steps:
[0029] Step S1, transforming the color space and geometric space of the scene picture, combining the original picture and the deformed picture together as the input layer of the deep convolutional neural network;
[0030] Step S2, constructing a structured network, and connecting three output branches to the last convolutional layer of the network. At each point on the final feature map, the first branch regression predicts the coordinate vectors of 12 candidate boxes, the second branch outputs the binary label vectors of the foreground and foreground of each candidate box, and the third branch outputs each candidate box Low-dimensional similar feature vectors of . Among them, on the basis of two or three channels, the networ...
PUM
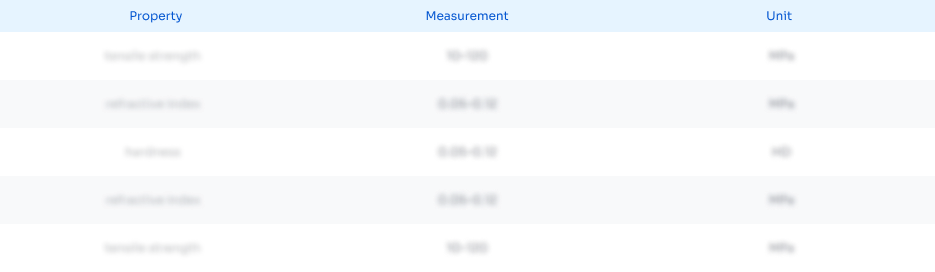
Abstract
Description
Claims
Application Information
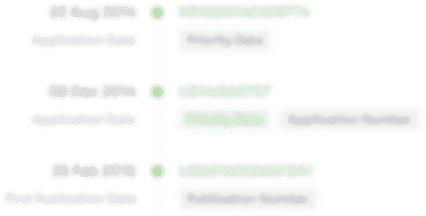
- R&D Engineer
- R&D Manager
- IP Professional
- Industry Leading Data Capabilities
- Powerful AI technology
- Patent DNA Extraction
Browse by: Latest US Patents, China's latest patents, Technical Efficacy Thesaurus, Application Domain, Technology Topic, Popular Technical Reports.
© 2024 PatSnap. All rights reserved.Legal|Privacy policy|Modern Slavery Act Transparency Statement|Sitemap|About US| Contact US: help@patsnap.com