Space target image restoration method based on convolutional auto-encoding convolutional Neural Network
A convolutional neural network and convolutional self-encoding technology, applied in the field of image processing, can solve the problems of poor neural network performance, unclear convolutional layer construction methods and reasons, and increased training difficulty, achieving excellent turbulent blur removal Ability, stable and clear display, excellent effect of anti-noise ability
- Summary
- Abstract
- Description
- Claims
- Application Information
AI Technical Summary
Problems solved by technology
Method used
Image
Examples
Embodiment Construction
[0036] In order to make the object, technical solution and advantages of the present invention clearer, the present invention will be further described in detail below in conjunction with the accompanying drawings and examples.
[0037] The convolutional autoencoder network extracts the low-dimensional data in the image set through encoding and compression, and then decodes and restores to the original image, so as to automatically learn the relevant features in the image sample. The convolutional autoencoder network first transforms the input data into a low-dimensional space, and then expands it to restore it to approximate the original image. This unsupervised learning method is often used to obtain the internal features of a series of related data sets, remove the redundant components of the input data, and obtain low-dimensional image features with certain robustness. The CAE neural network model that the present invention builds is as figure 1 .
[0038] Among them, f1, ...
PUM
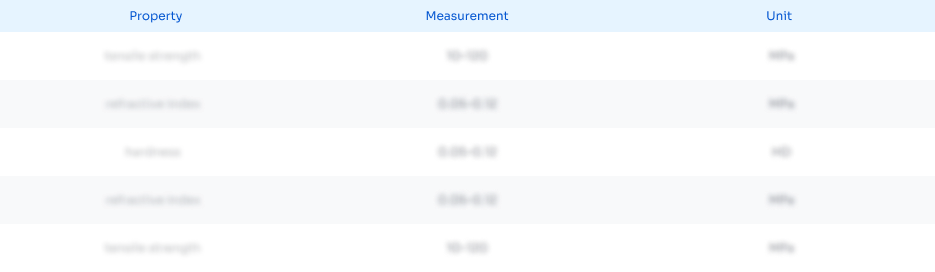
Abstract
Description
Claims
Application Information
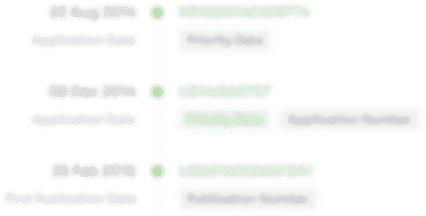
- R&D Engineer
- R&D Manager
- IP Professional
- Industry Leading Data Capabilities
- Powerful AI technology
- Patent DNA Extraction
Browse by: Latest US Patents, China's latest patents, Technical Efficacy Thesaurus, Application Domain, Technology Topic, Popular Technical Reports.
© 2024 PatSnap. All rights reserved.Legal|Privacy policy|Modern Slavery Act Transparency Statement|Sitemap|About US| Contact US: help@patsnap.com