Improved high-resolution remote sensing image classification method based on deep learning
A remote sensing image and deep learning technology, applied in the field of image processing, can solve the problems of complex feature extraction process, redundancy, poor displacement and rotation, etc.
- Summary
- Abstract
- Description
- Claims
- Application Information
AI Technical Summary
Problems solved by technology
Method used
Image
Examples
Embodiment Construction
[0086] The technical solutions of the present invention will be further described below in conjunction with the accompanying drawings and embodiments.
[0087] Such as figure 1 As shown, a further detailed description is as follows:
[0088] (1) Construct multi-category remote sensing image sample data sets, and make corresponding sample label sets, and divide remote sensing image sample data sets into training set Train and test set Test.
[0089] (1.1) Construct multi-category remote sensing image sample dataset Image=[Image 1 ,...,Image i ,...,Image N ], and make the corresponding sample label Label=[Label 1 ,...,Label i ,...,Label N ], where N represents N types of remote sensing images, Image i Indicates the set of i-th remote sensing images, Label i Represents the label set of the i-th category of remote sensing images, and the value of each category label is i. The present invention selects UCMerced_LandUse, a public data set of remote sensing scene images, for...
PUM
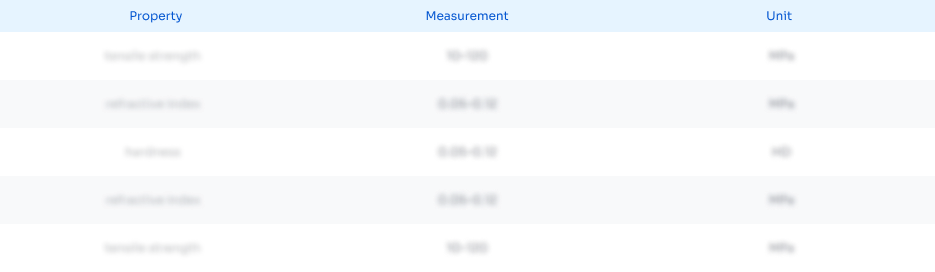
Abstract
Description
Claims
Application Information
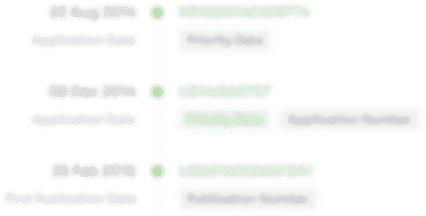
- R&D
- Intellectual Property
- Life Sciences
- Materials
- Tech Scout
- Unparalleled Data Quality
- Higher Quality Content
- 60% Fewer Hallucinations
Browse by: Latest US Patents, China's latest patents, Technical Efficacy Thesaurus, Application Domain, Technology Topic, Popular Technical Reports.
© 2025 PatSnap. All rights reserved.Legal|Privacy policy|Modern Slavery Act Transparency Statement|Sitemap|About US| Contact US: help@patsnap.com