Mechanical fault migration diagnosis method based on adaption sharing deep residual network (ASResNet)
A technology for mechanical faults and diagnosis methods, applied in neural learning methods, biological neural network models, neural architectures, etc., can solve the problem of high cost of manually labeling monitoring data, difficulty in training recognition accuracy fault intelligent diagnosis models, affecting the process of production and manufacturing, etc. question
- Summary
- Abstract
- Description
- Claims
- Application Information
AI Technical Summary
Problems solved by technology
Method used
Image
Examples
Embodiment Construction
[0049] The present invention will be described in further detail below in conjunction with the accompanying drawings.
[0050] Such as figure 1 As shown, a mechanical fault migration diagnosis method based on adaptive shared deep residual network, including the following steps:
[0051] 1) Obtain labeled monitoring data sets of laboratory equipment respectively and monitoring data sets of engineering equipment in are respectively the i-th sample in the laboratory equipment monitoring data set and its corresponding health status marker, is the i-th sample in the engineering equipment monitoring data set, and n is the number of minimum batch training samples;
[0052] 2) if figure 2 As shown, the i-th sample in the monitoring data set of laboratory equipment and engineering equipment is extracted by using the stacked residual unit in the Adaptive Shared Deep Residual Network (ASResNet) and Migration failure characteristics, that is, perform the following steps in s...
PUM
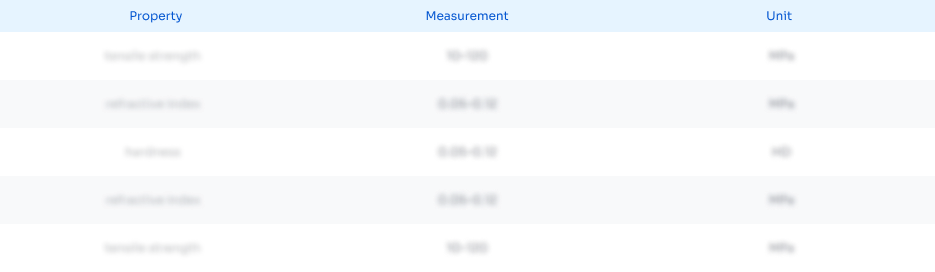
Abstract
Description
Claims
Application Information
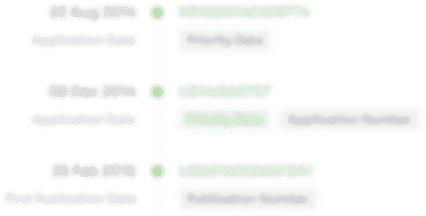
- R&D Engineer
- R&D Manager
- IP Professional
- Industry Leading Data Capabilities
- Powerful AI technology
- Patent DNA Extraction
Browse by: Latest US Patents, China's latest patents, Technical Efficacy Thesaurus, Application Domain, Technology Topic, Popular Technical Reports.
© 2024 PatSnap. All rights reserved.Legal|Privacy policy|Modern Slavery Act Transparency Statement|Sitemap|About US| Contact US: help@patsnap.com