Modeling and monitoring method of cutter abrasion loss on basis of residual error convolutional neural network
A technology of convolutional neural network and tool wear, applied in biological neural network models, neural learning methods, neural architectures, etc., can solve problems such as poor prediction results, damage to machine tools, affecting the quality of machined surfaces and dimensional accuracy, and achieve measurement process The effect of simplicity, fast data processing, and strong generalization performance
- Summary
- Abstract
- Description
- Claims
- Application Information
AI Technical Summary
Problems solved by technology
Method used
Image
Examples
Embodiment Construction
[0039] Embodiments of the present invention are described in detail below, and the embodiments are exemplary and intended to explain the present invention, but should not be construed as limiting the present invention.
[0040] A method for modeling and monitoring tool wear based on a residual convolutional neural network in this embodiment includes the following steps:
[0041] Step 1: According to the requirements of the tool wear experiment issued by the American PHM Society in 2008, namely figure 2 Install the force and acceleration and acoustic emission sensors in the form of the sensor, and do a good job of sensor calibration.
[0042] Three-axis force sensor, three-axis acceleration sensor and acoustic emission sensor are used; the force and acceleration sensors are placed on the workpiece or fixture, and the acoustic emission sensor is close to the side of the workpiece; the three-axis force sensor detects and converts the three-axis vibration force signal during proc...
PUM
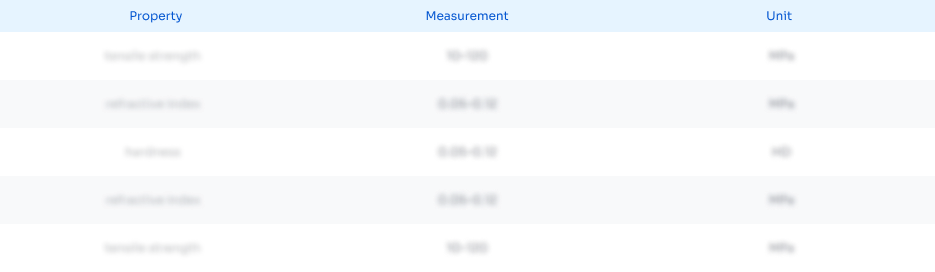
Abstract
Description
Claims
Application Information
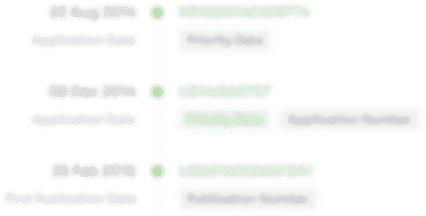
- R&D Engineer
- R&D Manager
- IP Professional
- Industry Leading Data Capabilities
- Powerful AI technology
- Patent DNA Extraction
Browse by: Latest US Patents, China's latest patents, Technical Efficacy Thesaurus, Application Domain, Technology Topic.
© 2024 PatSnap. All rights reserved.Legal|Privacy policy|Modern Slavery Act Transparency Statement|Sitemap