Aircraft electromechanical system fault identification method based on random convolutional neural network
A neural network and random convolution technology, applied in biological neural network models, neural architectures, electrical devices in testing and transportation, etc., to achieve the effect of reducing impact and solving reasonable conversion problems
- Summary
- Abstract
- Description
- Claims
- Application Information
AI Technical Summary
Problems solved by technology
Method used
Image
Examples
Embodiment 1
[0086] This example adopts the bearing failure data from the laboratory of Case Western Reserve University, and the test bearings adopt SKF bearings and NTN equivalent shafts. The faults in this experiment are single-point bearing faults, and the fault diameters are 0.1778mm, 0.3556mm, 0.5334mm, and 0.7112mm. The damage data of 0.1778mm, 0.3556mm, and 0.5334mm use SKF bearings, and other NTN equivalent bearings are used for testing. The fault types are divided into four types: normal, inner ring, outer ring, and rolling element. This example uses the data collected by the accelerometer at the drive end, and its sampling frequency is 12khz.
[0087] The experimental data set consists of 4 sets of driving end data (16×120,000 data points) under different motor speeds and load conditions. According to the type of fault, the degree of damage and the fault location, the fault state can be divided into 16 categories. The training sample set is 1600 samples under 1797rpm rotation s...
Embodiment 2
[0094] The test data in this example comes from the WS-ZHT1 multifunctional rotor test bench in our laboratory, including the multifunctional rotor test system developed by Beijing Pope Co., Ltd., the rotor bench experiment collector, and the SLM-500 eddy current signal conditioner. , Beijing Pope WS-ICP-6 vibration acceleration model conditioner, YG2003 DC speed control power supply, rotor test bench and Advantech 610H industrial computer. The rotor status is divided into 5 types: normal, unbalanced 1, unbalanced 2, rubbing, rubbing and unbalanced. Unbalance 1 and Unbalance 2 are under different speed and load conditions, and the sampling frequency is 10khz. The training sample set is 500 samples, the test sample is 200 samples, each sample contains 1024 sample points, and the 5 types of training samples are as follows: Figure 4 shown.
[0095] Perform short-time Fourier transform on 1024 points of each sample to obtain a time-frequency diagram of 32×32 power spectral dens...
PUM
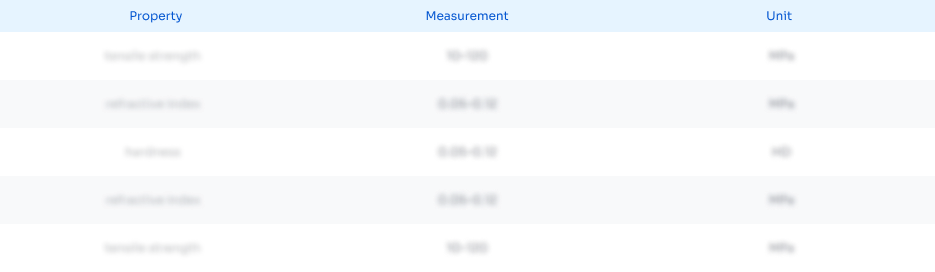
Abstract
Description
Claims
Application Information
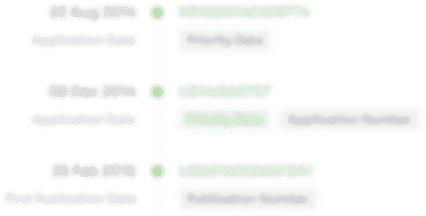
- R&D Engineer
- R&D Manager
- IP Professional
- Industry Leading Data Capabilities
- Powerful AI technology
- Patent DNA Extraction
Browse by: Latest US Patents, China's latest patents, Technical Efficacy Thesaurus, Application Domain, Technology Topic, Popular Technical Reports.
© 2024 PatSnap. All rights reserved.Legal|Privacy policy|Modern Slavery Act Transparency Statement|Sitemap|About US| Contact US: help@patsnap.com