Pedestrian detection method and system based on vehicle-mounted infrared video
A pedestrian detection and infrared technology, applied in the field of computer vision for pedestrian detection, can solve problems such as the influence of pedestrian accuracy
- Summary
- Abstract
- Description
- Claims
- Application Information
AI Technical Summary
Problems solved by technology
Method used
Image
Examples
Embodiment 1
[0064] see figure 1 As shown, embodiment 1 of the present invention provides a kind of pedestrian detection method based on vehicle-mounted infrared video, comprises the following steps:
[0065] S1. Obtain the infrared video through the vehicle-mounted infrared detector, and extract the color feature and gradient feature of the infrared video by using the integral map and channel features;
[0066] S2. Using the adaptive enhanced iterative algorithm Adaboost to train and screen the features extracted in step S1, detect pedestrians in the infrared video images, and obtain preliminary detection results;
[0067] S3. Using the Hungarian algorithm to calculate the preliminary detection results obtained in step S2, and obtain the initial trajectory sequence formed by the association of pedestrians in all images;
[0068] S4. Using the initial trajectory sequence obtained in step S3 as an initial value, the Mancles algorithm is used to calculate optimal trajectory correlation info...
Embodiment 2
[0070] On the basis of Embodiment 1, step S1 specifically includes the following steps:
[0071]The infrared video is obtained by the vehicle-mounted infrared detector, and the image of the infrared video is zoomed into an image pyramid; on different scales, using the integral map, the three color channel features L(I) of each frame image I after zooming, U(I), V(I), 6 gradient direction channel features G(I) 1[Θ=i] with angle Θ=i, 1 gradient amplitude channel feature||G(I)|| Extract respectively, and then perform normalization processing to obtain the integrated feature vector F representing the color feature and gradient feature of the frame image. The calculation formula of the integrated feature vector F is:
[0072] F={L(I),U(I),V(I),||G(I)||,G(I)·1[Θ=i]},
[0073]
Embodiment 3
[0075] On the basis of embodiment 2, step S2 specifically includes the following steps:
[0076] Let the input n training samples be: {(x 1 ,y 1 ),(x 2 ,y 2 ),…,(x n ,y n )}, where x i is the input training sample, y i ∈{0,1} represent positive samples and negative samples respectively, where the number of positive samples is l, the number of negative samples is m, n=l+m, l, m, and n are all positive integers; the adaptive enhanced iterative algorithm is adopted Adaboost, initialize the weight of each sample, train a weak classifier for detecting whether there are pedestrians in the image for each channel feature, calculate the weighted error rate of the weak classifier, adjust the weight according to the weak classifier with the smallest error rate, and iterate The selection process of the weak classifier is to synthesize the selected weak classifier into a strong classifier to detect whether there are pedestrians in the image; the strong classifier is used to train an...
PUM
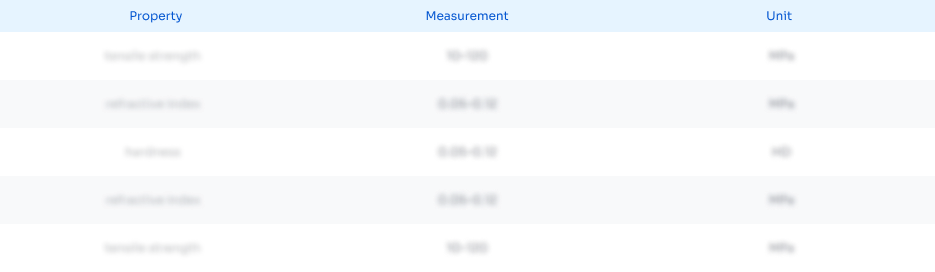
Abstract
Description
Claims
Application Information
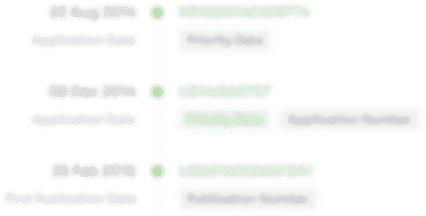
- R&D
- Intellectual Property
- Life Sciences
- Materials
- Tech Scout
- Unparalleled Data Quality
- Higher Quality Content
- 60% Fewer Hallucinations
Browse by: Latest US Patents, China's latest patents, Technical Efficacy Thesaurus, Application Domain, Technology Topic, Popular Technical Reports.
© 2025 PatSnap. All rights reserved.Legal|Privacy policy|Modern Slavery Act Transparency Statement|Sitemap|About US| Contact US: help@patsnap.com