Multi-dimensional time series forecasting method of total grain yield based on LSTM neural network
A neural network and time series technology, applied in neural learning methods, biological neural network models, prediction, etc., can solve problems such as inability to remember results, inability to solve long-distance dependencies, limited memory function, etc., to avoid the problem of gradient disappearance , good prediction effect, good applicability effect
- Summary
- Abstract
- Description
- Claims
- Application Information
AI Technical Summary
Problems solved by technology
Method used
Image
Examples
Embodiment Construction
[0029] The present invention will be further described below in conjunction with the accompanying drawings and specific embodiments.
[0030] The present embodiment discloses a multidimensional time series prediction method of total grain output based on LSTM neural network, comprising the following steps:
[0031] S01), preprocessing of input data
[0032] In engineering practice, the data we get is often incomplete, noisy, inconsistent, and repetitive, and the quality of input data in many deep learning algorithms determines the quality of the model in the training process, so we use data Before training the model, the data needs to be preprocessed. The process of data preprocessing is usually not fixed, it usually varies with different tasks and dataset properties.
[0033] In the field of deep learning, before using the training set to train the model, it is usually necessary to perform a normalized preprocessing operation on the data, mainly to limit the data to the ran...
PUM
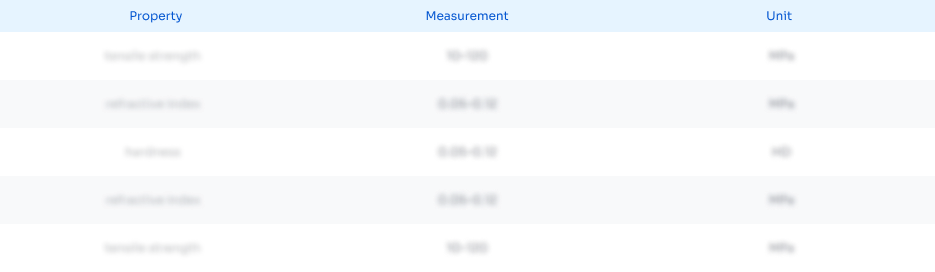
Abstract
Description
Claims
Application Information
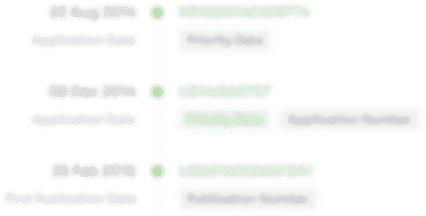
- R&D Engineer
- R&D Manager
- IP Professional
- Industry Leading Data Capabilities
- Powerful AI technology
- Patent DNA Extraction
Browse by: Latest US Patents, China's latest patents, Technical Efficacy Thesaurus, Application Domain, Technology Topic, Popular Technical Reports.
© 2024 PatSnap. All rights reserved.Legal|Privacy policy|Modern Slavery Act Transparency Statement|Sitemap|About US| Contact US: help@patsnap.com