Anomaly detection method for industrial control network signals based on depth learning structure
An industrial control network and deep learning technology, applied in the field of outlier detection in industrial control network data, can solve the problem of difficult detection of a small number of outliers, and achieve the effect of improving the ability
- Summary
- Abstract
- Description
- Claims
- Application Information
AI Technical Summary
Problems solved by technology
Method used
Image
Examples
specific Embodiment approach 1
[0024] Specific implementation mode one: refer to Figure 1 to Figure 4 Specifically illustrate this embodiment, the industrial control network signal anomaly detection method based on the deep learning structure described in this embodiment, the method includes the following steps:
[0025] Step 1. Select part of the data from the industrial control network data to mark as a training sample, perform data normalization and standardization operation on the training sample, obtain the normalized calibrated data, and use the data enhancement algorithm to normalize the normalized calibrated data. The calibration data adds some false sample values to form the detected data;
[0026] Step 2, input the normal data and the detected data into an autoencoder compression network for training, respectively obtain the spliced data of the normal data and the spliced data of the detected data;
[0027] Step 3, input the two spliced data into the comparison network and calculate the ...
specific Embodiment approach 2
[0044] Specific embodiment 2: This embodiment is a further description of the industrial control network signal anomaly detection algorithm based on the deep learning structure described in the specific embodiment 1. In this embodiment, in step 1, data normalization is performed on the training samples The formula for the normalization operation is:
[0045]
[0046] In the formula, is the mathematical expectation of the training sample data, σ x is the standard deviation of the training sample data, x k is the normalized calibrated data, x m is the calibrated data before normalization;
[0047] In step 1, the formula for adding some fake samples to the normalized calibrated data using the data enhancement algorithm is:
[0048]
[0049]
[0050] In the formula, λ is a value in the range of (0,1), (x i ,y i ),(x j ,y j ) are two samples randomly obtained from the normalized calibrated data; is the value and label of the generated "fake samples".
specific Embodiment approach 3
[0051] Specific embodiment three: This embodiment is a further description of the industrial control network signal anomaly detection algorithm based on the deep learning structure described in specific embodiment one. In this embodiment, in step three, the two spliced data Input to the comparison network and calculate the distance between the normal data and the detected data through the calculation of the deep neural network is as follows:
[0052] Using the parameter θ c neural network to learn the difference L(f n ,f a ; θ c ), the distance f(x) between the normal data and the detected data is obtained as:
[0053] f(x)=L([x n ,z n ],[x a ,z a ]; θ c ) Formula 3,
[0054] where f n represented by normal data X n and the compressed value Z for normal data n Splicing data of normal data obtained by splicing and combining, f a Indicates that by the new detected data X a and the detected data compression value Z a The spliced data of the detected data obtain...
PUM
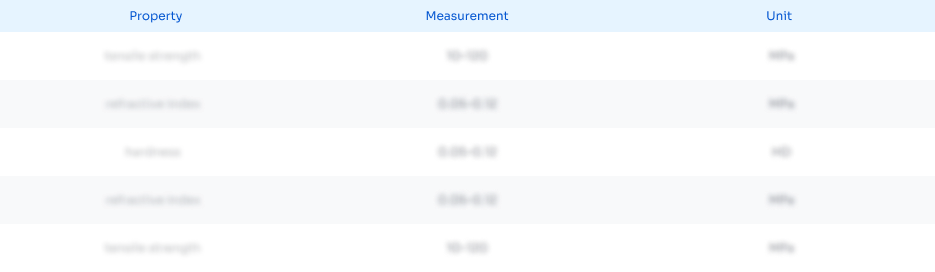
Abstract
Description
Claims
Application Information
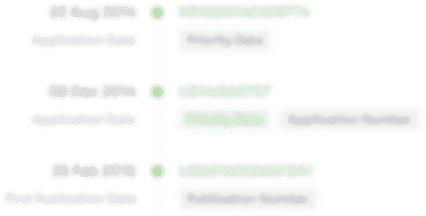
- R&D
- Intellectual Property
- Life Sciences
- Materials
- Tech Scout
- Unparalleled Data Quality
- Higher Quality Content
- 60% Fewer Hallucinations
Browse by: Latest US Patents, China's latest patents, Technical Efficacy Thesaurus, Application Domain, Technology Topic, Popular Technical Reports.
© 2025 PatSnap. All rights reserved.Legal|Privacy policy|Modern Slavery Act Transparency Statement|Sitemap|About US| Contact US: help@patsnap.com