An Objective Evaluation Method of Speech Quality Based on Deep Neural Network
A technology of deep neural network and objective evaluation method, applied in speech analysis, instruments, etc., can solve the problems of inability to evaluate variable-speed speech, wideband signal quality evaluation of narrowband signal, and inability to target speech signal quality evaluation, etc., to achieve accurate evaluation results Effect
- Summary
- Abstract
- Description
- Claims
- Application Information
AI Technical Summary
Problems solved by technology
Method used
Image
Examples
Embodiment 1
[0093] To test the network model, the present invention makes predictions on test set data. The test set uses the test part of the TIMIT speech library, which contains a total of 1672 pure speech sentences. The speakers are different from the network training set data. The test set data generates noisy speech with the same signal-to-noise ratio as the training set, but its noise The kind is different from the noise samples used in the training set. In the experiment, we predict the test data under each signal-to-noise ratio, and compare the data with the scores obtained by the actual PESQ algorithm. The results are as follows (the data in the table are all under the same signal-to-noise ratio. average value):
[0094] Table 1 Distribution table of predicted results and actual PESQ results
[0095] SNR / dB -40 -30 -20 -10 0 10 20 30 40 forecast result 1.15 1.13 1.18 1.37 1.89 2.56 3.32 3.92 4.31 PESQ results 0.94 0.94 0.99 1.27 1.81 ...
Embodiment 2
[0098] PESQ algorithm can't objectively evaluate the pitch-shifted voice quality even if there is a reference signal. For this reason, in order to prove that the present invention is applicable to this kind of situation, a section of noisy voice with a signal-to-noise ratio of 10 decibels and 3.5 seconds is selected. The signal is evaluated, and its time-domain waveform diagram is as follows Figure 4 As shown, the 1.5x speed variable speed speech signal is as follows Figure 5 As shown, the corresponding pure speech signals of the two are as follows Image 6 with Figure 7 shown. The score given by the actual PESQ algorithm before the speed change is 2.18, and the score predicted by the algorithm is 2.26. After the speed change, the actual PESQ score is 2.07, and the score predicted by the algorithm is 2.16, which has not changed, indicating that the present invention is applicable to this situation.
Embodiment 3
[0100] Considering the generalization ability of the present invention to Chinese speech, the present invention adds part of the THCH30 corpus on the basis of the original training set. In view of the fact that in the implementation example 1, the actual PESQ score has no significant difference for the noisy speech score below -20db, the SNR range selected in this example is -30-40db. The final prediction results of TIMIT test voice and THCH30 test voice are as follows Figure 8 with Figure 9 shown.
PUM
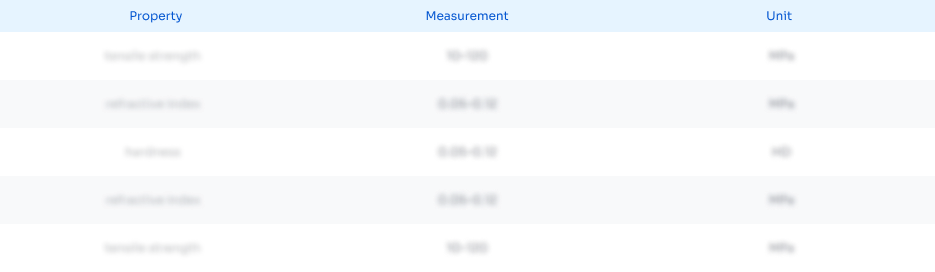
Abstract
Description
Claims
Application Information
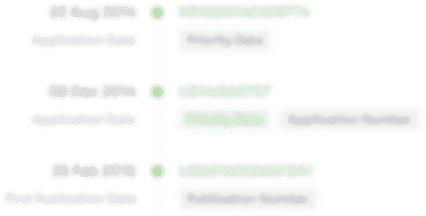
- R&D Engineer
- R&D Manager
- IP Professional
- Industry Leading Data Capabilities
- Powerful AI technology
- Patent DNA Extraction
Browse by: Latest US Patents, China's latest patents, Technical Efficacy Thesaurus, Application Domain, Technology Topic, Popular Technical Reports.
© 2024 PatSnap. All rights reserved.Legal|Privacy policy|Modern Slavery Act Transparency Statement|Sitemap|About US| Contact US: help@patsnap.com