Method and device for establishing multi-attribute recognition model and method for multi-attribute recognition
A recognition model and multi-attribute technology, applied in the field of multi-attribute recognition model establishment, can solve problems such as difficulty in learning to obtain effective feature representations and classifiers, complex implementation of region recommendations, ignoring attribute correlations, etc., to achieve easy addition and deletion of attributes , highly flexible, easy to modify effects
- Summary
- Abstract
- Description
- Claims
- Application Information
AI Technical Summary
Problems solved by technology
Method used
Image
Examples
Embodiment Construction
[0031] The specific implementation manners of the present invention will be further described in detail below in conjunction with the accompanying drawings and embodiments. The following examples are used to illustrate the present invention, but are not intended to limit the scope of the present invention.
[0032] The embodiment of the present invention provides a method and device for establishing a multi-attribute recognition model, and a multi-attribute recognition method. Different from the prior art, a network structure is added to realize the potential spatial region relationship between attributes and semantic recognition. The relationship is extracted, and only the annotation information of the picture is used to realize supervised learning, which can realize accurate identification of multiple attributes, and the training cost is low and the efficiency is high. The establishment method of the multi-attribute recognition model can also be understood as a training meth...
PUM
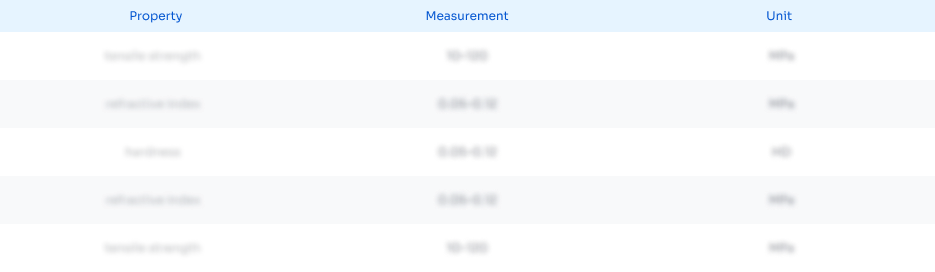
Abstract
Description
Claims
Application Information
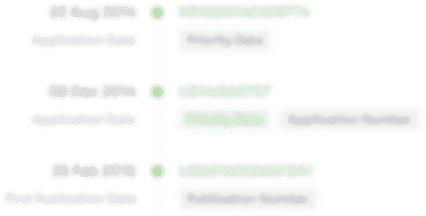
- R&D
- Intellectual Property
- Life Sciences
- Materials
- Tech Scout
- Unparalleled Data Quality
- Higher Quality Content
- 60% Fewer Hallucinations
Browse by: Latest US Patents, China's latest patents, Technical Efficacy Thesaurus, Application Domain, Technology Topic, Popular Technical Reports.
© 2025 PatSnap. All rights reserved.Legal|Privacy policy|Modern Slavery Act Transparency Statement|Sitemap|About US| Contact US: help@patsnap.com