PM2.5 concentration prediction method based on stack self-encoding and support vector regression
A technology of support vector regression and stacked self-encoding, which is applied in prediction, instrumentation, biological neural network model, etc., can solve problems such as inability to obtain higher accuracy, cannot exceed one or two hidden layers, etc., and achieve excellent prediction performance. Effect
- Summary
- Abstract
- Description
- Claims
- Application Information
AI Technical Summary
Problems solved by technology
Method used
Image
Examples
Embodiment Construction
[0031] The technical solutions of the present invention will be described in detail below in conjunction with the accompanying drawings.
[0032] In the field of artificial intelligence, the ANN optimization algorithm is the most widely used algorithm with excellent performance, but it can never go beyond one or two hidden layers in non-convex optimization problems, resulting in the inability to learn deep networks to achieve higher accuracy. In order to overcome this problem, a SAE with multiple sparse autoencoders as hidden layers is introduced to extract the features of the dataset, and then the SVR model is used to improve the prediction performance. Based on this, the present invention proposes a PM2.5 concentration prediction method combining stacked autoencoder and support vector regression.
[0033] Such as figure 1 As shown, the PM2.5 concentration prediction method that combines stacked autoencoder and support vector regression includes the following steps:
[0034...
PUM
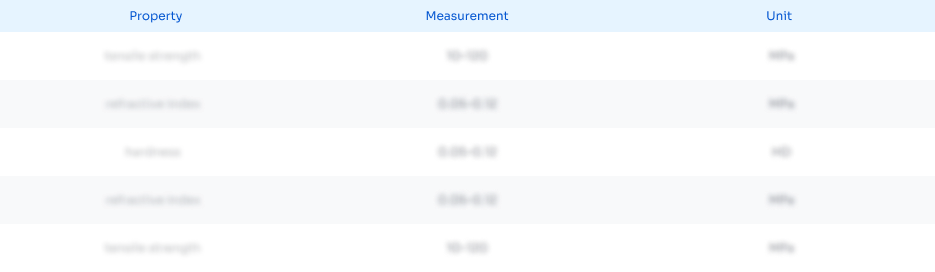
Abstract
Description
Claims
Application Information
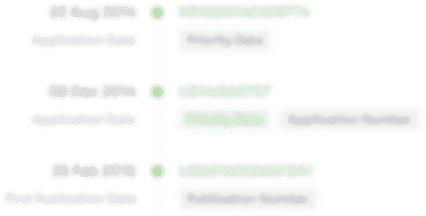
- R&D
- Intellectual Property
- Life Sciences
- Materials
- Tech Scout
- Unparalleled Data Quality
- Higher Quality Content
- 60% Fewer Hallucinations
Browse by: Latest US Patents, China's latest patents, Technical Efficacy Thesaurus, Application Domain, Technology Topic, Popular Technical Reports.
© 2025 PatSnap. All rights reserved.Legal|Privacy policy|Modern Slavery Act Transparency Statement|Sitemap|About US| Contact US: help@patsnap.com