XGBoost prediction method of intelligent parameter optimization module
A technology for optimizing modules and prediction methods, which is applied in the fields of financial data prediction, model parameter optimization and machine learning prediction. scene-specific effects
- Summary
- Abstract
- Description
- Claims
- Application Information
AI Technical Summary
Problems solved by technology
Method used
Image
Examples
Embodiment Construction
[0025] Step 1. Apply the present invention to a data set of 300 binary classifications of liver diseases with a feature number of 6. The features of this type of data are independent and identically distributed, which meets the major prerequisites of the algorithm of the present invention.
[0026] Step 2. Since it is hoped that the classification of the XGBoost model can be as smooth and clear as possible, and the error is small, the parameter group selects the learning rate learningRate, the number of base classifiers nEstimators, the maximum depth maxDepth, the minimum weight of leaf nodes minChildWeight, the node splitting coefficient gammaValue, The seven parameters of random sampling ratio subSample and random sampling column number colSampleByTree are optimized and adjusted by genetic methods.
[0027] Step 3. Using the genetic method, construct an optimization module for optimizing the XGBoost model parameter group, optimize the parameters of the XGBoost model, and impr...
PUM
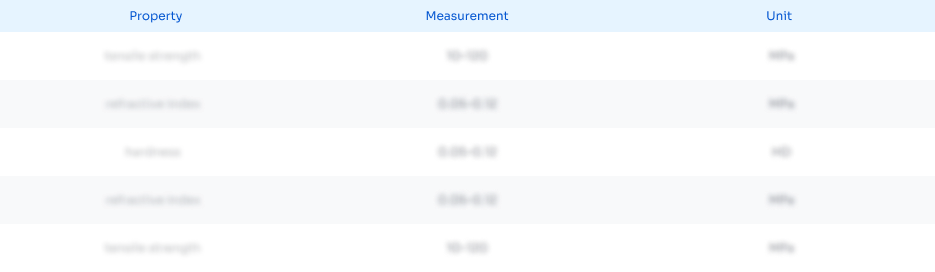
Abstract
Description
Claims
Application Information
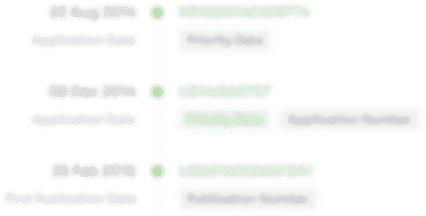
- R&D Engineer
- R&D Manager
- IP Professional
- Industry Leading Data Capabilities
- Powerful AI technology
- Patent DNA Extraction
Browse by: Latest US Patents, China's latest patents, Technical Efficacy Thesaurus, Application Domain, Technology Topic, Popular Technical Reports.
© 2024 PatSnap. All rights reserved.Legal|Privacy policy|Modern Slavery Act Transparency Statement|Sitemap|About US| Contact US: help@patsnap.com