Chip image super-resolution reconstruction method based on depth learning
A technology of super-resolution reconstruction and deep learning, which is applied in the field of image super-resolution reconstruction and hardware Trojan detection. It can solve the problems of poor chip image effect, expensive, low image resolution, etc., and achieve the goal of improving the effect of super-resolution reconstruction. Effect
- Summary
- Abstract
- Description
- Claims
- Application Information
AI Technical Summary
Problems solved by technology
Method used
Image
Examples
Embodiment Construction
[0024] refer to figure 1 , the specific implementation steps of the present invention are as follows:
[0025] Step 1: Divide the image set.
[0026] Take photos of a large number of microarray images to collect microarray images, and randomly divide the collected microarray images into image sets to be processed {y (1) ,y (2) ,...y (l) ,...,y (N)} and the test set {t (1) ,t (2) ,...t (d) ...,t (M)}, where y (l) is the lth image in the image set to be processed, l=1,2,...,N,t (d) is the dth image in the test set, d=1,2,...,M, N is the number of images in the image set to be processed, and M is the number of images in the test set.
[0027] Step 2: Obtain a training data set according to the images in the image set to be processed.
[0028] refer to figure 2 , the specific implementation of this step is as follows:
[0029] (2a) In order to increase the final training data set, it is first necessary to expand the number of images in the image set to be processed t...
PUM
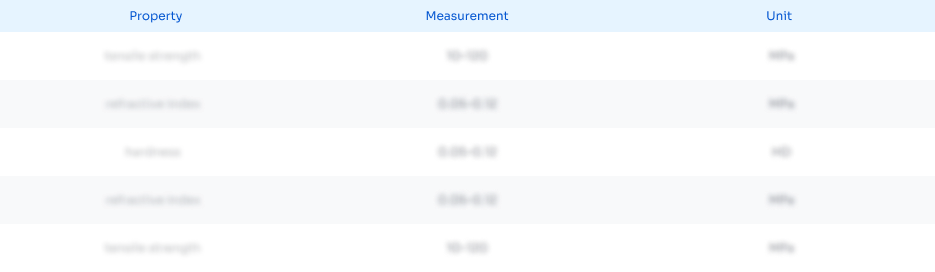
Abstract
Description
Claims
Application Information
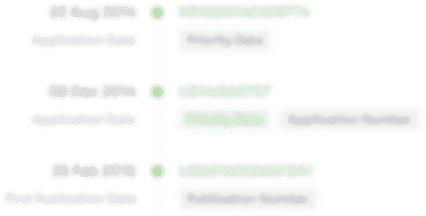
- R&D
- Intellectual Property
- Life Sciences
- Materials
- Tech Scout
- Unparalleled Data Quality
- Higher Quality Content
- 60% Fewer Hallucinations
Browse by: Latest US Patents, China's latest patents, Technical Efficacy Thesaurus, Application Domain, Technology Topic, Popular Technical Reports.
© 2025 PatSnap. All rights reserved.Legal|Privacy policy|Modern Slavery Act Transparency Statement|Sitemap|About US| Contact US: help@patsnap.com