An object detection method based on semantic segmentation enhancement
A technology of semantic segmentation and object detection, applied in neural learning methods, biological neural network models, instruments, etc., can solve the problems of low detection accuracy and achieve the effect of improving accuracy and safety
- Summary
- Abstract
- Description
- Claims
- Application Information
AI Technical Summary
Problems solved by technology
Method used
Image
Examples
Embodiment Construction
[0023] The present invention will be further described below in conjunction with the accompanying drawings.
[0024] figure 2 Examples of conventional deep convolutional neural networks applied to object detection are described. Specifically, this type of method inputs the original image into the designed convolutional neural network, directly regresses to obtain the coordinates of all categories of objects, and outputs the corresponding categories of objects. The features on which the predictions are based are category-independent features, that is, the features cannot explicitly reflect the characteristics of each type of object.
[0025] image 3 An example of the application of the deep convolutional neural network based on semantic segmentation enhancement proposed by the present invention to object detection is described. Specifically, this deep neural network consists of three main parts: backbone subnetwork, segmentation subnetwork and detection subnetwork. The ba...
PUM
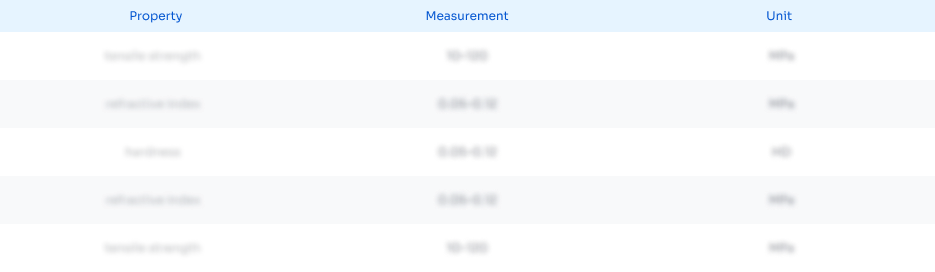
Abstract
Description
Claims
Application Information
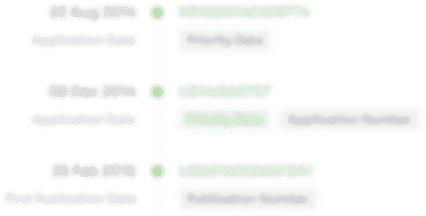
- R&D Engineer
- R&D Manager
- IP Professional
- Industry Leading Data Capabilities
- Powerful AI technology
- Patent DNA Extraction
Browse by: Latest US Patents, China's latest patents, Technical Efficacy Thesaurus, Application Domain, Technology Topic, Popular Technical Reports.
© 2024 PatSnap. All rights reserved.Legal|Privacy policy|Modern Slavery Act Transparency Statement|Sitemap|About US| Contact US: help@patsnap.com