A multi-dimensional information fusion method for transformer fault diagnosis based on depth learning
A transformer fault and deep learning technology, which is applied to instruments, biological neural network models, character and pattern recognition, etc., can solve problems such as relying on labeled samples, achieve fast parameter convergence, high recognition accuracy, and strong expression ability Effect
- Summary
- Abstract
- Description
- Claims
- Application Information
AI Technical Summary
Problems solved by technology
Method used
Image
Examples
Embodiment Construction
[0023] The specific implementation of the present invention will be further described below in conjunction with drawings and examples, but the implementation and protection of the present invention are not limited thereto. Achieved with technology.
[0024] Such as figure 1 , a deep learning-based multi-dimensional information transformer fault diagnosis method proposed in this example, including the following steps:
[0025] (1) Determine the deep learning method based on the restricted Boltzmann machine. The RBM model consists of a visible layer and a hidden layer. There are two states of network neurons: inactive state and active state. They are represented by 0 and 1 respectively. It is stipulated that there is no self-connection between the neurons in the layer, and the neurons between the layers are fully connected with each other. The model structure is as follows: figure 2 shown.
[0026] no v and n h Respectively represent the number of neurons contained in the...
PUM
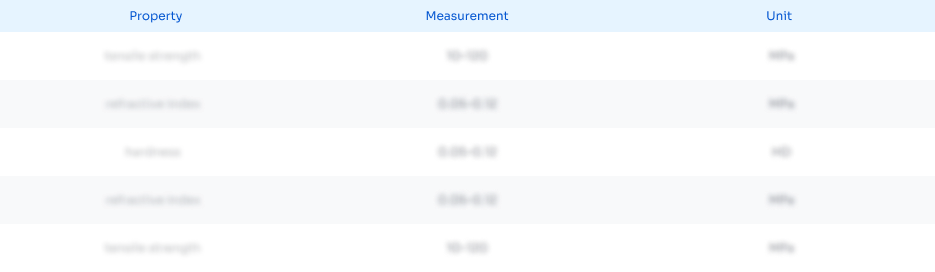
Abstract
Description
Claims
Application Information
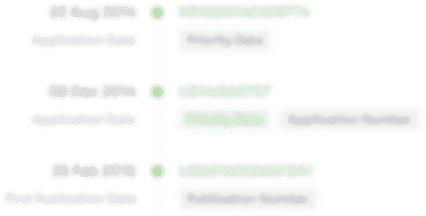
- Generate Ideas
- Intellectual Property
- Life Sciences
- Materials
- Tech Scout
- Unparalleled Data Quality
- Higher Quality Content
- 60% Fewer Hallucinations
Browse by: Latest US Patents, China's latest patents, Technical Efficacy Thesaurus, Application Domain, Technology Topic, Popular Technical Reports.
© 2025 PatSnap. All rights reserved.Legal|Privacy policy|Modern Slavery Act Transparency Statement|Sitemap|About US| Contact US: help@patsnap.com