A convolution neural network method for differentiation of hepatocellular carcinoma
A convolutional neural network and liver cancer technology, applied in the super-field, can solve the problems of missed diagnosis, time-consuming, etc., and achieve the effect of accurately distinguishing results, avoiding strong observation, and avoiding shortcomings and missed diagnosis.
- Summary
- Abstract
- Description
- Claims
- Application Information
AI Technical Summary
Problems solved by technology
Method used
Image
Examples
Embodiment Construction
[0015] The technical solution of the present invention will be specifically described below in conjunction with the accompanying drawings.
[0016] The present invention provides a method for distinguishing the differentiation grade of liver cancer by a convolutional neural network, which is used to distinguish the MPM image data of liver cancer biopsy samples or samples sent for inspection after liver cancer resection, including the following process:
[0017] 1. Prepare data;
[0018] 217 MPM images of liver cancer were collected by a multiphoton microscope system (eg figure 2 ), set the excitation wavelength of the system to 810nm, and select an upright 20× objective lens (0.8 NA) to scan the sample. Set up 2 receiving channels, one at 370-419 nm, marked in green, for collecting SHG signals; the other at 420-700 nm, marked in red, for detecting TPEF signals. The size of a single image is 425.10×425.10 μm2, and the pixels are 512×512. Diagnosed by experienced pathologist...
PUM
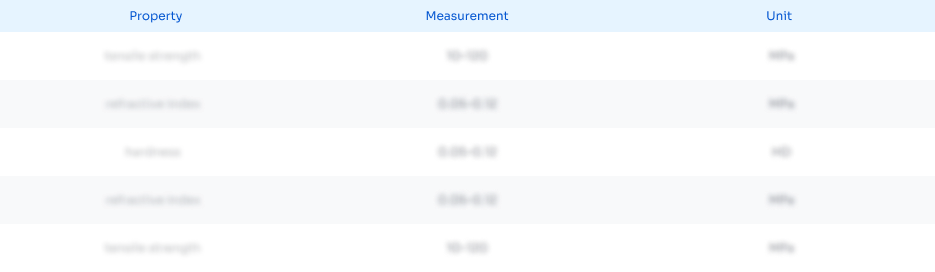
Abstract
Description
Claims
Application Information
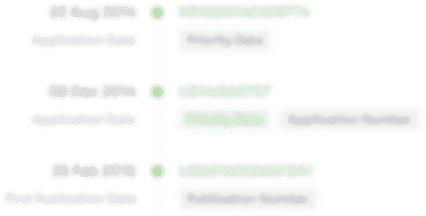
- R&D Engineer
- R&D Manager
- IP Professional
- Industry Leading Data Capabilities
- Powerful AI technology
- Patent DNA Extraction
Browse by: Latest US Patents, China's latest patents, Technical Efficacy Thesaurus, Application Domain, Technology Topic, Popular Technical Reports.
© 2024 PatSnap. All rights reserved.Legal|Privacy policy|Modern Slavery Act Transparency Statement|Sitemap|About US| Contact US: help@patsnap.com