LSTM loop neural network model and network attack identification method based on the model
A cyclic neural network and network attack technology, which is applied in the field of network security, can solve problems such as manslaughtering normal business traffic, false positives or false positives, rule conflicts, etc., and achieve the effect of avoiding feature engineering, less feature coding, and smaller weight files
- Summary
- Abstract
- Description
- Claims
- Application Information
AI Technical Summary
Problems solved by technology
Method used
Image
Examples
Embodiment 1
[0036] refer to Figure 1~3 As shown, the present invention provides a kind of LSTM cycle neural network model that is used for network attack category recognition, and the establishment process of described LSTM cycle neural network model comprises the following steps:
[0037] S100: Collect multiple network request data in text format as a training data set, and set a label category for each network request data according to the content of the network request data.
[0038] The multiple refers to a large amount of data in this field, and the larger the amount of data, the more accurate the final model.
[0039] The network request data can be divided into multiple categories according to the type of network attack, mainly divided into normal network request data and attack category network request data, in this embodiment, mainly to distinguish SQL injection attack and XSS attack, therefore, the The above label categories include three types, namely category 1: normal netwo...
Embodiment 2
[0077] A network attack identification method, based on the LSTM cyclic neural network model for network attack category identification described in Embodiment 1, the method includes: preprocessing the network request data to obtain digital sequence data of a preset length, using The LSTM cyclic neural network model identifies the probability of the label category of the digital sequence data to obtain the category probability of each label category, and uses the label category corresponding to the dimension with the largest probability value in the category probability as the label category of the network request data. Choose whether to intercept according to the predicted results.
[0078] The identification of whether to intercept can specifically set a probability threshold, and intercept when the probability of category 2 or category 3 is greater than or equal to the probability threshold, otherwise, do not intercept.
[0079] In this embodiment, the probability threshold...
Embodiment 3
[0081] Such as Figure 5 As shown, a network attack identification system, based on the network attack identification method described in Embodiment 2, includes a data input unit, a data conversion unit, an LSTM model unit and a decision unit, and the data input unit receives the network request data Send to the data conversion unit, after the data conversion unit preprocesses and converts the network request data, after obtaining the digital sequence data of a preset length, sends the digital sequence data to the LSTM model unit, and the LSTM model unit The probability of the label category of the digital sequence data is identified, and the category probability of each label category is output to the decision-making unit, and the decision-making unit judges whether the probability that the data is an attack category is greater than or equal to a preset probability threshold, and if so, If it is intercepted, otherwise, it is not intercepted.
[0082] The attack category is a...
PUM
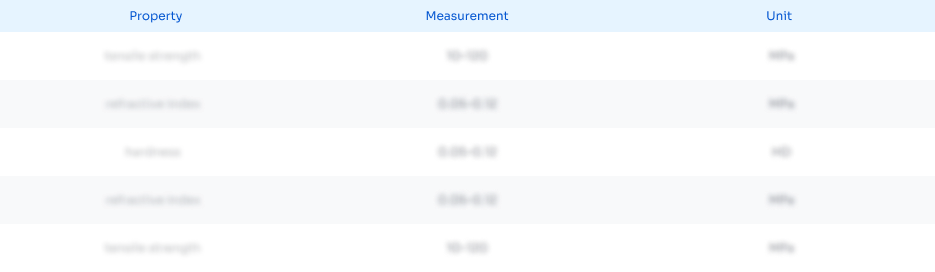
Abstract
Description
Claims
Application Information
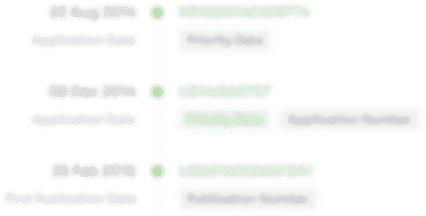
- R&D Engineer
- R&D Manager
- IP Professional
- Industry Leading Data Capabilities
- Powerful AI technology
- Patent DNA Extraction
Browse by: Latest US Patents, China's latest patents, Technical Efficacy Thesaurus, Application Domain, Technology Topic.
© 2024 PatSnap. All rights reserved.Legal|Privacy policy|Modern Slavery Act Transparency Statement|Sitemap