Local learning regularization-based depth matrix decomposition method and image clustering method
A matrix decomposition and local learning technology, applied in the field of image processing, can solve the problem that non-negative matrices are difficult to meet the multi-attribute properties of data, and achieve the effect of efficient use
- Summary
- Abstract
- Description
- Claims
- Application Information
AI Technical Summary
Problems solved by technology
Method used
Image
Examples
Embodiment Construction
[0026] In order to more clearly illustrate the embodiments of the present invention or the technical solutions in the prior art, the specific implementation manners of the present invention will be described below with reference to the accompanying drawings. Obviously, the accompanying drawings in the following description are only some embodiments of the present invention, and those skilled in the art can obtain other accompanying drawings based on these drawings and obtain other implementations.
[0027] For deep semi-non-negative matrix factorization (Deep Semi-NMF), the original dataset matrix Y that does not limit the positive and negative internal elements is given ± Decompose into (m+1) factors, so that the product of these (m+1) factors is as close as possible to the original data set matrix Y ± approximately equal. Under the limitation of non-negative coefficient matrix, deep semi-non-negative matrix regularization keeps information unchanged as much as possible, an...
PUM
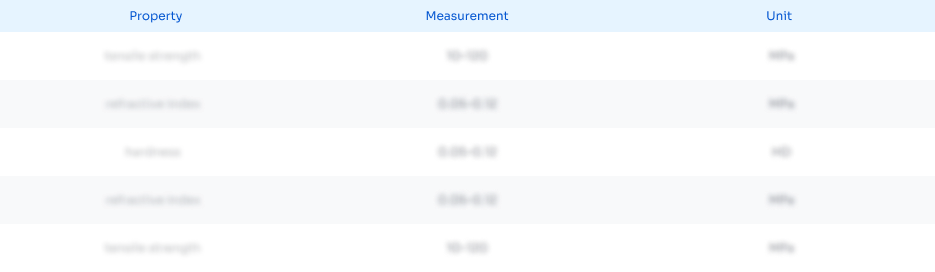
Abstract
Description
Claims
Application Information
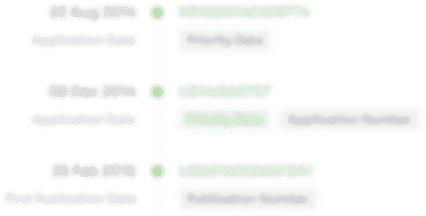
- R&D
- Intellectual Property
- Life Sciences
- Materials
- Tech Scout
- Unparalleled Data Quality
- Higher Quality Content
- 60% Fewer Hallucinations
Browse by: Latest US Patents, China's latest patents, Technical Efficacy Thesaurus, Application Domain, Technology Topic, Popular Technical Reports.
© 2025 PatSnap. All rights reserved.Legal|Privacy policy|Modern Slavery Act Transparency Statement|Sitemap|About US| Contact US: help@patsnap.com