A method for efficiently tensioning a fully connected neural network
A neural network and fully connected technology, which is applied in the field of highly efficient tensorized fully connected neural networks, can solve the problems of sensitive initialization of network weight parameters and unstable network accuracy, and achieve the effect of improving classification accuracy and reducing the number of parameters.
- Summary
- Abstract
- Description
- Claims
- Application Information
AI Technical Summary
Problems solved by technology
Method used
Image
Examples
Embodiment Construction
[0039] The present invention will be further described below in conjunction with specific embodiment:
[0040] See attached figure 1 As shown, a method for efficiently tensorizing a fully connected neural network described in this embodiment includes the following steps:
[0041] S1: Input the fully connected layer of the neural network into the vector x'∈R N Represented in higher-order tensor form: The spatial information in the input of the fully connected layer can be preserved to improve the classification accuracy of the neural network; after the vector is expressed as a tensor, its elements have not changed, but the dimension has changed to n 1 ×n 2 ×…×n n ; For ease of description and visualization of tensors, this embodiment uses a circle to represent a tensor, the number of line segments on the circle represents the number of dimensions of the tensor, and the numbers next to the line segments represent the size of the dimension. input tensor graphics such as ...
PUM
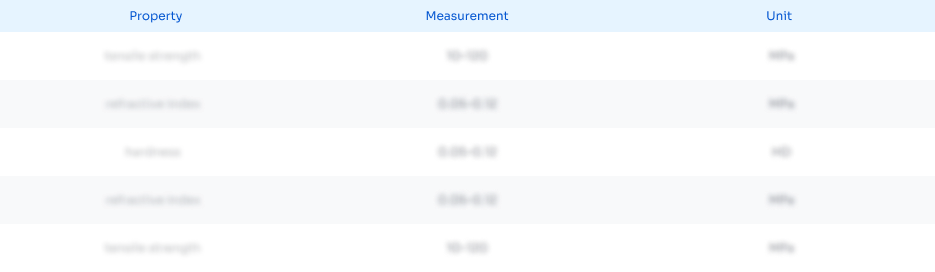
Abstract
Description
Claims
Application Information
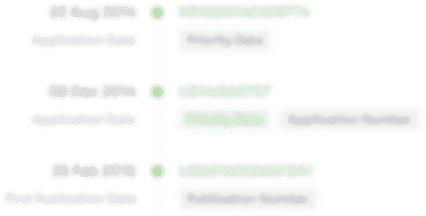
- R&D Engineer
- R&D Manager
- IP Professional
- Industry Leading Data Capabilities
- Powerful AI technology
- Patent DNA Extraction
Browse by: Latest US Patents, China's latest patents, Technical Efficacy Thesaurus, Application Domain, Technology Topic, Popular Technical Reports.
© 2024 PatSnap. All rights reserved.Legal|Privacy policy|Modern Slavery Act Transparency Statement|Sitemap|About US| Contact US: help@patsnap.com