Micro-power-grid energy storage scheduling method and device based on deep Q-value network (DQN) reinforcement learning
A technology of reinforcement learning and energy storage scheduling, which is applied in the direction of circuit devices, AC network circuits, AC network load balancing, etc., can solve the problems of insufficient estimation ability of deep Q value network, insufficient stability and accuracy of learning objectives, etc., and achieve estimation ability Strong, stable learning objectives
- Summary
- Abstract
- Description
- Claims
- Application Information
AI Technical Summary
Problems solved by technology
Method used
Image
Examples
specific Embodiment 1
[0056] Such as figure 1 As shown, the embodiment of the present invention provides a microgrid energy storage scheduling method based on deep Q-value network reinforcement learning, including:
[0057] Step S101 is established to establish a microgrid model;
[0058] Training step S102, according to the microgrid model, use the deep Q value network reinforcement learning algorithm to carry out artificial intelligence training;
[0059] Calculation step S103, according to the input parameter characteristic value, calculate and obtain the battery operation strategy of microgrid energy storage dispatch.
[0060] Such as figure 2 As shown, preferably, the micro-grid model can be provided with sequentially connected battery pack energy storage systems, photovoltaic power generation systems, power loads and control devices, and the power loads and control devices are connected to the distribution network through a common connection point. The electricity price information of the...
specific Embodiment 2
[0116] Such as Figure 6 As shown, the embodiment of the present invention provides a microgrid energy storage scheduling device based on deep Q-value network reinforcement learning, including:
[0117] Build module 201, used to build a microgrid model;
[0118] The training module 202 is used to perform artificial intelligence training using a deep Q value network reinforcement learning algorithm according to the microgrid model;
[0119] The calculation module 203 is used to calculate the battery operation strategy for energy storage scheduling of the microgrid according to the input parameter characteristic values.
[0120] The embodiment of the present invention uses a deep Q value network to schedule and manage the energy of the microgrid. The intelligent body interacts with the environment to determine the optimal energy storage scheduling strategy, and controls the operation mode of the battery in a constantly changing environment, based on the dynamic decision of the ...
PUM
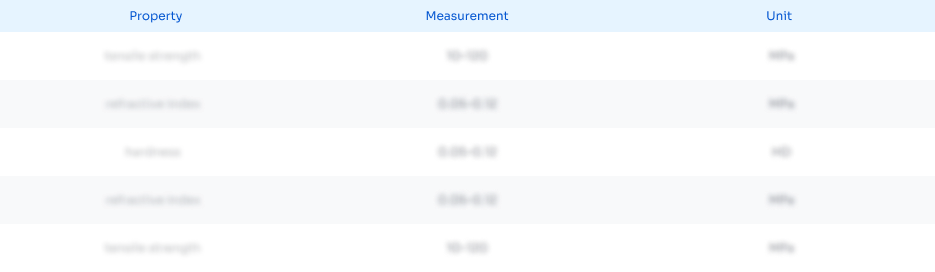
Abstract
Description
Claims
Application Information
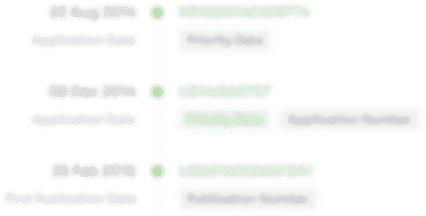
- R&D Engineer
- R&D Manager
- IP Professional
- Industry Leading Data Capabilities
- Powerful AI technology
- Patent DNA Extraction
Browse by: Latest US Patents, China's latest patents, Technical Efficacy Thesaurus, Application Domain, Technology Topic, Popular Technical Reports.
© 2024 PatSnap. All rights reserved.Legal|Privacy policy|Modern Slavery Act Transparency Statement|Sitemap|About US| Contact US: help@patsnap.com