A method and apparatus for processing knowledge map based on semi-supervised embedded representation model
A technology of knowledge graph and embedded representation, which is applied in the field of knowledge graph processing based on semi-supervised embedded representation model, which can solve problems such as difficult to predict whether there is a connection, performance limitations, etc.
- Summary
- Abstract
- Description
- Claims
- Application Information
AI Technical Summary
Problems solved by technology
Method used
Image
Examples
Embodiment 1
[0046] From the perspective of graph theory, the knowledge graph is essentially a graph, which can be expressed as G=(V,E), where V represents the set of vertices in the graph, and V=v 1 ,...,v n , E represents the edge set in the graph, E=e 1 ,....,e n . For the knowledge graph, each vertex v represents an entity, and each edge e represents the relationship between two entities.
[0047] The geometric meanings of the first-order proximity and the second-order proximity in the present invention are as follows:
[0048] First-order proximity: The first-order proximity describes the similarity between a pair of entities. For any pair of entities, if v i And v j There is an edge between v i And v j There is a relationship between v i And v j The first-order proximity between them is positive. Otherwise, v i And v j The first-order proximity between is 0.
[0049] According to the above definition, it is easy to know that the key to calculating the first-order proximity is to calculate...
Embodiment 2
[0114] In this embodiment, the method described in embodiment 1 is used to realize a typical application of knowledge graph-entity classification, and the objects of the application are two popular corpora of FB15K and WIN18. First, pre-process the two popular corpora to remove all entities that are not described by the associated triples. The parameters of the preprocessed corpus are shown in Table 1.
[0115] Table 1
[0116] Corpus
#Rel
#Ent
#Valid
#Test
FB15K
1336
14885
472860
50000
57800
WIN18
18
40100
140975
5000
5000
[0117] In order to form a comparison, several current most advanced methods are selected for comparison in this embodiment: including TransE, TransD, DKRL (CNN), Jointly (LSTM) and Jointly (ALSTM). The parameters used for training of all models are the parameter settings used to obtain the best performance.
[0118] The task of entity classification is a multi-label classification task aimed at predicting entity types. Almost every entity has a typ...
Embodiment 3
[0123] In this embodiment, the method described in embodiment 1 is used to realize another typical application of knowledge graph—link prediction. The target of the application is still the two popular corpora of FB15K and WIN18, and the two popular corpora are used in the same way as in embodiment 2. Two popular corpora are preprocessed, and the parameters of the two popular corpora after preprocessing are shown in Table 1.
[0124] Link prediction is a typical task of perfecting the triple (h, r, t) of the knowledge graph, where h or t is missing, that is, given (h, r) predicts t. This task places more emphasis on ranking a set of candidate entities from the knowledge graph. This embodiment uses two metrics as evaluation indicators, namely MeanRank and Hits@10, where MeanRank is the average of the correct number of entities or relationships, and Hits@10 is the ratio of the top p effective entities or relationships in the prediction. In this embodiment, p=10 is set for the enti...
PUM
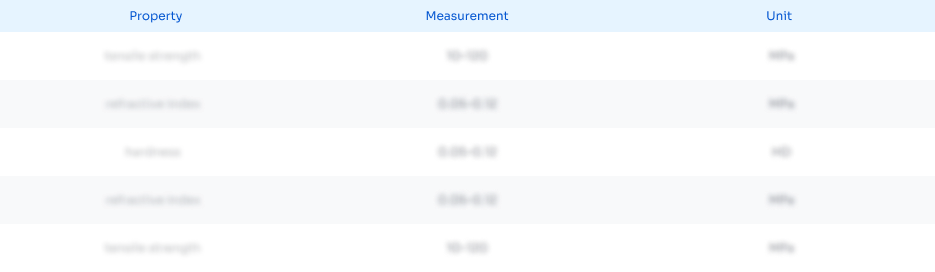
Abstract
Description
Claims
Application Information
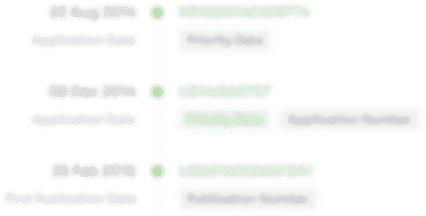
- R&D
- Intellectual Property
- Life Sciences
- Materials
- Tech Scout
- Unparalleled Data Quality
- Higher Quality Content
- 60% Fewer Hallucinations
Browse by: Latest US Patents, China's latest patents, Technical Efficacy Thesaurus, Application Domain, Technology Topic, Popular Technical Reports.
© 2025 PatSnap. All rights reserved.Legal|Privacy policy|Modern Slavery Act Transparency Statement|Sitemap|About US| Contact US: help@patsnap.com