A millimeter wave imaging dangerous goods detection method based on FPGA and depth learning
A millimeter wave imaging and dangerous goods technology, applied in character and pattern recognition, biological neural network models, instruments, etc., can solve the problem of inability to accurately extract the irregular shape and outline of dangerous goods, and achieve fine concurrent operation granularity and concurrent execution efficiency. , fast processing speed, good function and customizability advantage
- Summary
- Abstract
- Description
- Claims
- Application Information
AI Technical Summary
Problems solved by technology
Method used
Image
Examples
Embodiment Construction
[0038] The embodiments of the present invention are described in detail below. This embodiment is implemented on the premise of the technical solution of the present invention, and provides a detailed implementation manner and a specific operation process, but the protection scope of the present invention is not limited to the following implementation. example.
[0039] like figure 1 and figure 2 As shown, this embodiment includes two parts: offline model training and online detection, which are described as follows:
[0040] 1. Offline training of deep learning network model part:
[0041] Using the image semantic segmentation annotation tool labelme, the outlines of all dangerous objects in each image are marked separately as the input of the deep learning network.
[0042] The convolutional neural network used to extract features has a total of 8 layers, including 5 convolutional layers and 3 max-pooling layers. Normalize the input image data, adjust the image size to ...
PUM
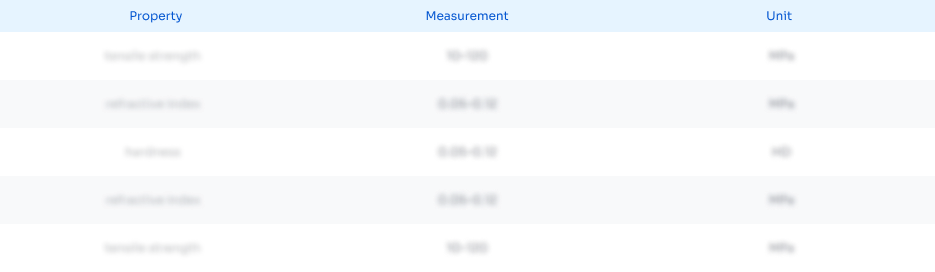
Abstract
Description
Claims
Application Information
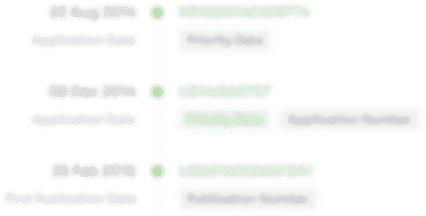
- Generate Ideas
- Intellectual Property
- Life Sciences
- Materials
- Tech Scout
- Unparalleled Data Quality
- Higher Quality Content
- 60% Fewer Hallucinations
Browse by: Latest US Patents, China's latest patents, Technical Efficacy Thesaurus, Application Domain, Technology Topic, Popular Technical Reports.
© 2025 PatSnap. All rights reserved.Legal|Privacy policy|Modern Slavery Act Transparency Statement|Sitemap|About US| Contact US: help@patsnap.com