A Depth Prediction Method of Monocular Image Based on Neural Network
A neural network and prediction method technology, applied in the field of image depth prediction, can solve problems such as not fully considering global and local features, and achieve the effects of reducing the amount of calculation parameters, saving calculation costs, and low calculation complexity
- Summary
- Abstract
- Description
- Claims
- Application Information
AI Technical Summary
Problems solved by technology
Method used
Image
Examples
Embodiment Construction
[0026] The present invention will be further described in detail below in conjunction with the accompanying drawings and embodiments.
[0027] A neural network-based monocular image depth prediction method proposed by the present invention, its overall realization block diagram is as follows figure 1 As shown, it includes two processes of training phase and testing phase;
[0028] The specific steps of the described training phase process are:
[0029] Step 1_1: Select Q original monocular images and the real depth images corresponding to each original monocular image, and form a training set, record the qth original monocular image in the training set as {I q (i,j)|1≤i≤W,1≤j≤H}, combine the training set with {I q (i,j)|1≤i≤W, 1≤j≤H} corresponding to the real depth image is recorded as Among them, Q is a positive integer, Q≥200, such as taking Q=4000, q is a positive integer, 1≤q≤Q, 1≤i≤W, 1≤j≤H, W means {I q (i,j)|1≤i≤W,1≤j≤H} and The width of H means {I q (i,j)|1≤i≤W...
PUM
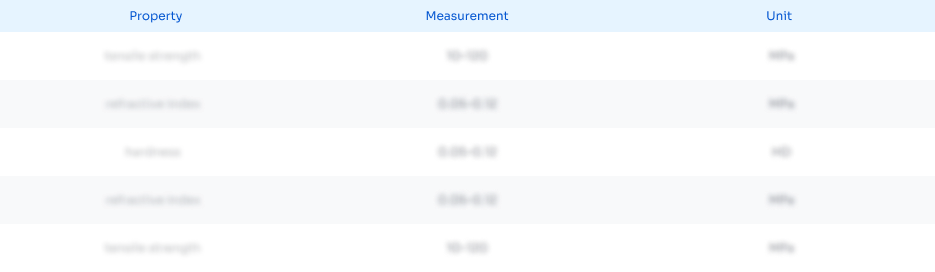
Abstract
Description
Claims
Application Information
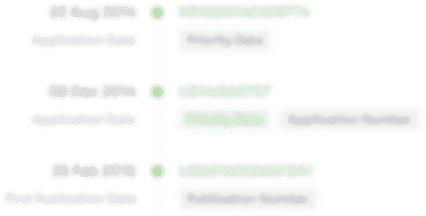
- R&D Engineer
- R&D Manager
- IP Professional
- Industry Leading Data Capabilities
- Powerful AI technology
- Patent DNA Extraction
Browse by: Latest US Patents, China's latest patents, Technical Efficacy Thesaurus, Application Domain, Technology Topic.
© 2024 PatSnap. All rights reserved.Legal|Privacy policy|Modern Slavery Act Transparency Statement|Sitemap