An automatic archive classification method based on an extreme learning machine
An extreme learning machine and automatic classification technology, applied in neural learning methods, text database clustering/classification, semantic analysis, etc., can solve the problems of insufficient efficiency and low dimensionality of archive text classification, improve classification accuracy, and simplify network training Effect
- Summary
- Abstract
- Description
- Claims
- Application Information
AI Technical Summary
Problems solved by technology
Method used
Image
Examples
Embodiment 1
[0052] Example 1: see figure 1 . The file automatic classification method based on the extreme learning machine of the present invention mainly includes two stages: a model learning stage and a model running stage. Each stage contains four modules: preprocessing module, text feature extraction module, low-level feature and middle-level feature fusion module, and archive classification module based on extreme learning machine. The text feature extraction module contains two sub-modules: the bottom-level feature extraction module and the middle-level feature autonomous learning module. The steps are as follows:
[0053] (1) Training sample preprocessing: normalize the text training sample set used for model learning to remove information irrelevant to the task;
[0054] (2) Low-level feature extraction of text training samples: The samples processed by the preprocessing module are sent to the bottom-level feature extraction module to extract the bottom-level features of the text. I...
Embodiment 2
[0064] Example 2: see figure 1 , figure 2 . The file automatic classification method of the extreme learning machine of this embodiment will further describe the technical solution of pooling in step (3) and step (6). The process of this step is as follows:
[0065] (1) Assuming that the archive file contains x words, and t words are left after the bottom-level feature extraction, this text is expressed as , Where the word vector of each word is , Each word vector has k-dimensional features;
[0066] (2) Divide the word vector in the text T into N parts to form N word vector groups, and each group corresponds to t / N word vectors;
[0067] (3) Perform the following operations for each word vector group: accumulate all word vectors in the group, and finally each word vector group will form a feature vector v(z), the dimension of the feature vector is also k;
[0068] (4) Concatenate the feature vectors of N word vector groups to get the feature vector of the entire document, as show...
Embodiment 3
[0071] Example three: see figure 1 , image 3 . In this embodiment, based on the automatic file classification method of the extreme learning machine, the technical solution of step (10) is further described in detail. Step (10) The details of the classification of the sample files to be determined are as follows:
[0072] The algorithm consists of the following steps:
[0073] (1) Extract the bottom-level features, middle-level features and fusion features of the text samples separately;
[0074] (2) Send the three types of features to the trained file classification model based on low-level features, the trained file classification model based on middle-level features, and the trained file classification model based on fusion features;
[0075] (3) Add the output result vectors of the three classification models (each dimension of the vector corresponds to one of the file categories, and the value of each dimension represents the probability that the text sample belongs to the file...
PUM
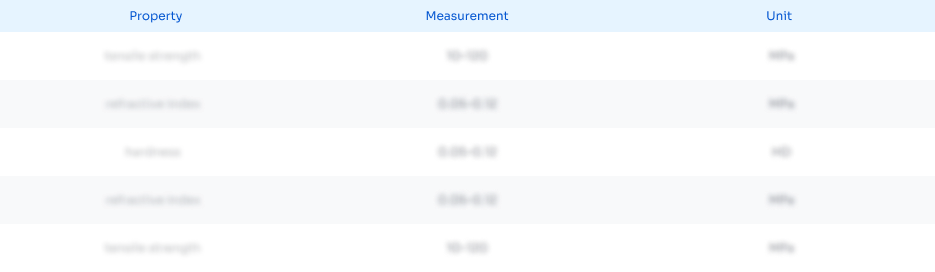
Abstract
Description
Claims
Application Information
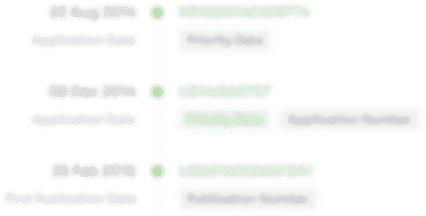
- R&D
- Intellectual Property
- Life Sciences
- Materials
- Tech Scout
- Unparalleled Data Quality
- Higher Quality Content
- 60% Fewer Hallucinations
Browse by: Latest US Patents, China's latest patents, Technical Efficacy Thesaurus, Application Domain, Technology Topic, Popular Technical Reports.
© 2025 PatSnap. All rights reserved.Legal|Privacy policy|Modern Slavery Act Transparency Statement|Sitemap|About US| Contact US: help@patsnap.com