An unsupervised industrial system anomaly detection method based on deep transfer learning
An industrial system, transfer learning technology, applied in the fields of data mining, deep learning and neural networks
- Summary
- Abstract
- Description
- Claims
- Application Information
AI Technical Summary
Problems solved by technology
Method used
Image
Examples
Embodiment Construction
[0017] The unsupervised industrial system anomaly detection method based on deep transfer learning of the present invention comprises the following steps:
[0018] 1) Collect sensor data;
[0019] 2) Data preprocessing;
[0020] 3) Divide the dataset,
[0021] 4) Model building;
[0022] 5) Model training;
[0023] 6) Model testing;
[0024] 7) Determine whether there is a fault.
[0025] When a new machine is added to the industrial system, repeat steps 1) to 6) to get a new model, and then redeploy the model.
[0026] The above step 2) data preprocessing divides sensor data into three categories, the first category has a certain range of data; the second category is data that increases with time; the third category is data with only fixed state quantities; The data in a certain range of change is processed by normalization, which compresses the range of the training set data to [0,1]; the data that increases with time adopts differential processing, and the differentia...
PUM
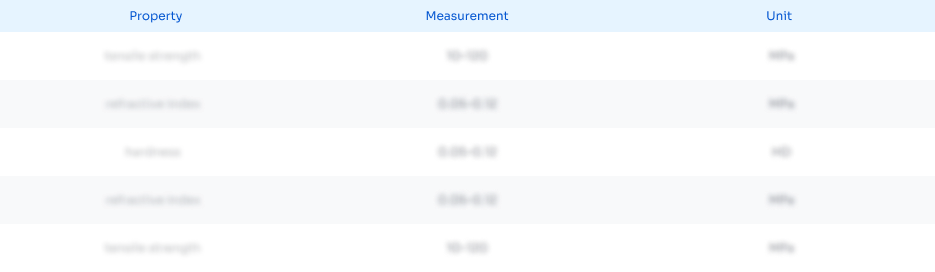
Abstract
Description
Claims
Application Information
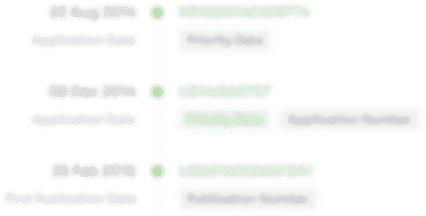
- R&D Engineer
- R&D Manager
- IP Professional
- Industry Leading Data Capabilities
- Powerful AI technology
- Patent DNA Extraction
Browse by: Latest US Patents, China's latest patents, Technical Efficacy Thesaurus, Application Domain, Technology Topic, Popular Technical Reports.
© 2024 PatSnap. All rights reserved.Legal|Privacy policy|Modern Slavery Act Transparency Statement|Sitemap|About US| Contact US: help@patsnap.com