Seasonal commodity demand prediction method based on time sequence decomposition
A time series and demand forecasting technology, applied in instruments, computational models, biological models, etc., can solve problems such as the inapplicability of the SARIMAX model and the long calculation cycle.
- Summary
- Abstract
- Description
- Claims
- Application Information
AI Technical Summary
Problems solved by technology
Method used
Image
Examples
Embodiment Construction
[0040] In order to make the object and effect of the present invention clearer, the commodity demand forecasting method based on time series decomposition will be described in detail below.
[0041]Similar to other demand forecasting models, the input of the model disclosed in the present invention is the historical sales records of commodities. Since the sales records are not continuous in time, before using the model, the date of the historical sales records must be completed to make it date continuous. In the time series of , missing values can be filled with 0 (representing no sales on this day). In addition, the model also needs weather data in the same time period and in the same region. The weather data is required to be continuous in time and include at least two dimensions: date and daily average temperature. It is worth noting that when the model predicts each day in the test period, it is necessary to select a length n 2 The training data and length n 3 (n 3 >n...
PUM
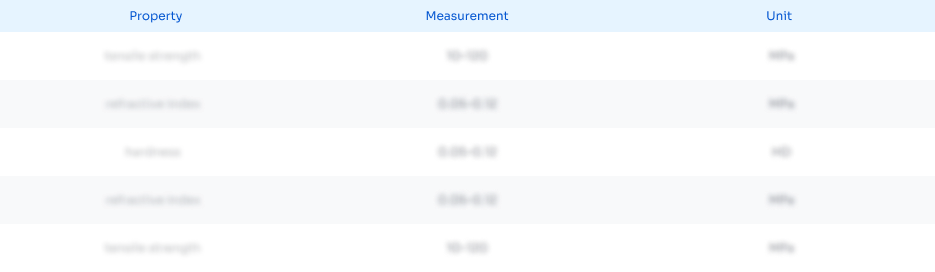
Abstract
Description
Claims
Application Information
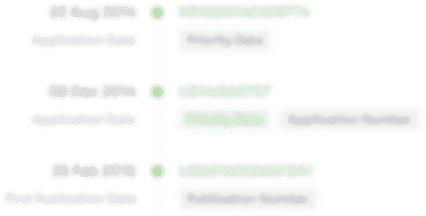
- R&D Engineer
- R&D Manager
- IP Professional
- Industry Leading Data Capabilities
- Powerful AI technology
- Patent DNA Extraction
Browse by: Latest US Patents, China's latest patents, Technical Efficacy Thesaurus, Application Domain, Technology Topic, Popular Technical Reports.
© 2024 PatSnap. All rights reserved.Legal|Privacy policy|Modern Slavery Act Transparency Statement|Sitemap|About US| Contact US: help@patsnap.com