OLTC mechanical fault diagnosis method based on sample entropy and SVM
A technology for mechanical faults and diagnosis methods, applied in the testing of mechanical parts, computer parts, character and pattern recognition, etc. Good anti-noise and anti-interference ability, good OLTC fault diagnosis effect
- Summary
- Abstract
- Description
- Claims
- Application Information
AI Technical Summary
Problems solved by technology
Method used
Image
Examples
Embodiment Construction
[0060] The present invention will be further described below in conjunction with the accompanying drawings.
[0061] Such as figure 1 As shown, a OLTC mechanical fault diagnosis method based on sample entropy and SVM includes the following steps:
[0062] Step 1: Place the acceleration vibration sensor on the top cover of the OLTC to collect vibration signals in various states.
[0063] Step 2: Decompose the vibration signals in various states through EEMD (Ensemble Empirical Mode Decomposition) to obtain the component IMF, and take the first k (4≤k≤6) IMF components for further processing;
[0064] Step 3: Calculate the sample entropy of the selected IMF component;
[0065] Step 4: For the training data set, use the calculated sample entropy as the feature vector, input the SVM for training, and obtain the SVM classifier, input the SampEn value of the IMF component of the test sample into the SVM classifier, and obtain the test from the output of the SVM classifier The run...
PUM
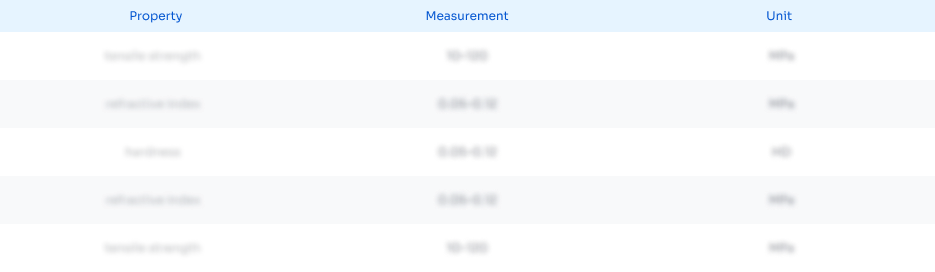
Abstract
Description
Claims
Application Information
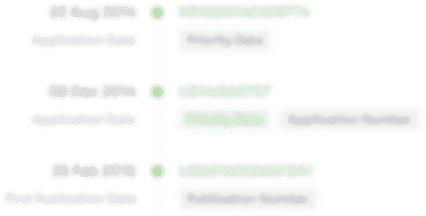
- R&D Engineer
- R&D Manager
- IP Professional
- Industry Leading Data Capabilities
- Powerful AI technology
- Patent DNA Extraction
Browse by: Latest US Patents, China's latest patents, Technical Efficacy Thesaurus, Application Domain, Technology Topic, Popular Technical Reports.
© 2024 PatSnap. All rights reserved.Legal|Privacy policy|Modern Slavery Act Transparency Statement|Sitemap|About US| Contact US: help@patsnap.com