Few-sample learning classifier construction method based on unbalanced data
A technology of sample learning and construction methods, which is applied in the directions of instruments, calculations, character and pattern recognition, etc., can solve the problem that the target data cannot be processed in a unified binary classification, and achieve the effect of stable classification performance and good classification results
- Summary
- Abstract
- Description
- Claims
- Application Information
AI Technical Summary
Problems solved by technology
Method used
Image
Examples
Embodiment
[0058] 1) Experimental data:
[0059]KEEL and NASA are open source machine learning data warehouses. The experiment randomly selected 14 data sets from these two machine learning warehouses for analysis. They are CM1, Appendicitis (Appe), Bupa, KC1, Ionosphere (Iono), Mammographic (Mamm), MW1, Phoneme (Phon), PC1, Ring, Sonar, Twonorm (Twon), Spambase (Spam) and Wisconsin (Wisc). They have different feature dimensions, the smallest feature attribute is 5, and the largest feature attribute is 60; their class imbalance ratios are also different, the smallest class imbalance ratio is 2, and the largest class imbalance ratio is 16. And each data set has a limited number of instances, the minimum number of instances is 106, and the maximum number of instances is 7400. Therefore, it is difficult for traditional machine learning classification algorithms to train effective data classification models from the above data sets.
[0060] 2) Comparison method:
[0061] The benchmark co...
PUM
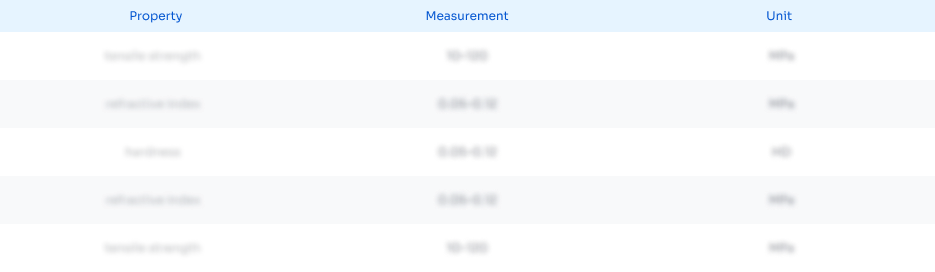
Abstract
Description
Claims
Application Information
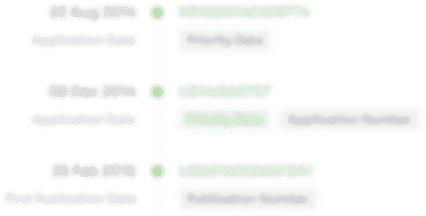
- R&D Engineer
- R&D Manager
- IP Professional
- Industry Leading Data Capabilities
- Powerful AI technology
- Patent DNA Extraction
Browse by: Latest US Patents, China's latest patents, Technical Efficacy Thesaurus, Application Domain, Technology Topic, Popular Technical Reports.
© 2024 PatSnap. All rights reserved.Legal|Privacy policy|Modern Slavery Act Transparency Statement|Sitemap|About US| Contact US: help@patsnap.com