A multi-target fast fuzzy clustering color image segmentation method based on semi-supervised learning and histogram statistics
A technique of histogram statistics and semi-supervised learning, applied in the field of image processing, can solve problems such as insufficient supervision information, too large number of clustering categories, and difficulty in obtaining class label information, so as to ensure diversity and evolutionary stability, reduce Calculation amount and effect of shortening processing time
- Summary
- Abstract
- Description
- Claims
- Application Information
AI Technical Summary
Problems solved by technology
Method used
Image
Examples
Embodiment Construction
[0032] The present invention will be described in detail below in conjunction with specific embodiments. The following examples will help those skilled in the art to further understand the present invention, but do not limit the present invention in any form. It should be noted that those skilled in the art can make several modifications and improvements without departing from the concept of the present invention. These all belong to the protection scope of the present invention.
[0033] The invention provides a multi-objective fast fuzzy clustering color image segmentation method based on semi-supervised learning and histogram statistics, such as figure 1 As shown, it specifically includes the following steps:
[0034] Step 1. Input the color image to be segmented and pairwise constraint information.
[0035] Step 2. Set the initial population number of the image to be segmented pop ,The maximum number of iterations and the maximum number of clusters K .
[0036] St...
PUM
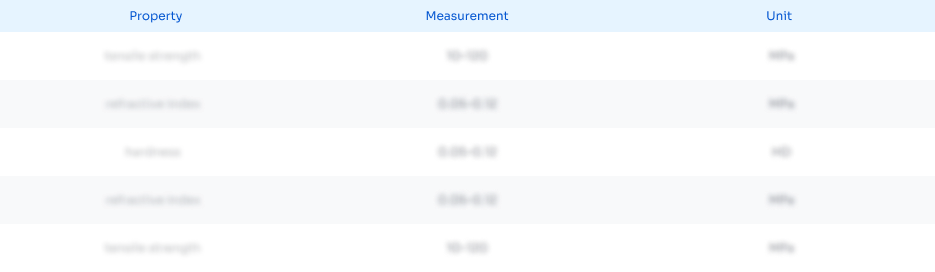
Abstract
Description
Claims
Application Information
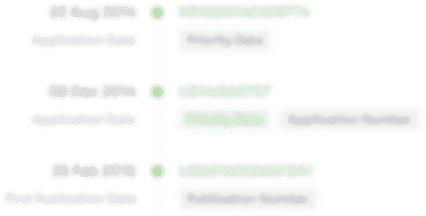
- R&D
- Intellectual Property
- Life Sciences
- Materials
- Tech Scout
- Unparalleled Data Quality
- Higher Quality Content
- 60% Fewer Hallucinations
Browse by: Latest US Patents, China's latest patents, Technical Efficacy Thesaurus, Application Domain, Technology Topic, Popular Technical Reports.
© 2025 PatSnap. All rights reserved.Legal|Privacy policy|Modern Slavery Act Transparency Statement|Sitemap|About US| Contact US: help@patsnap.com