Auxiliary diagnosis system for large-scale medical records based on deep learning
A deep learning and auxiliary diagnosis technology, applied in medical automation diagnosis, computer-aided medical procedures, informatics, etc., can solve problems such as difficult multi-feature multi-category medical data set prediction
- Summary
- Abstract
- Description
- Claims
- Application Information
AI Technical Summary
Problems solved by technology
Method used
Image
Examples
Embodiment
[0036] Medical records: Chief complaint: "headache and fever", age: "male", diagnosis: "acute upper respiratory tract infection".
[0037] Vectorization: Chief Complaint: "23,89,76,99", Age: "2", Diagnosis: "305".
[0038] splice to fixed length: "0,0,...,0,23,89,76,99,0,0,0,2,0,...,0", diagnostics: "0,...,0,1,0, ...,0"
[0039] The above is a sequence, and other training medical records are converted into sequences according to the above method, which are used to train the cyclic neural network model.
[0040] Symptoms corresponding to "acute upper respiratory tract infection": "headache, fever, runny nose, sneezing, ...".
[0041] Artificially generated medical records: "headache, runny nose, sneezing, fever", diagnosis: "acute upper respiratory infection".
[0042]vectorization: '[2,1,…,3],[3,4,…,1],[5,2,…,3],[2,3,…,1]', diagnostics: '305' .
[0043] Splice to fixed length: "[0,…,0],[0,…,0],…,[0,…,0],[2,1,…,3],[3,4,…,1 ],[5,2,…,3],[2,3,…,1]”, diagnosis: “0,…,0,1,0,…,0...
PUM
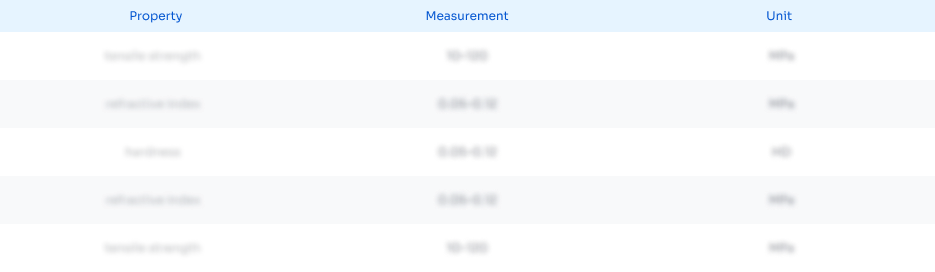
Abstract
Description
Claims
Application Information
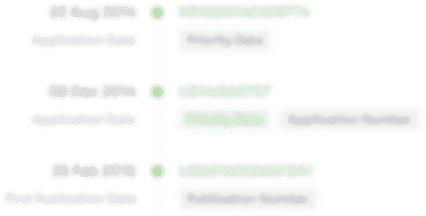
- R&D Engineer
- R&D Manager
- IP Professional
- Industry Leading Data Capabilities
- Powerful AI technology
- Patent DNA Extraction
Browse by: Latest US Patents, China's latest patents, Technical Efficacy Thesaurus, Application Domain, Technology Topic, Popular Technical Reports.
© 2024 PatSnap. All rights reserved.Legal|Privacy policy|Modern Slavery Act Transparency Statement|Sitemap|About US| Contact US: help@patsnap.com