Road driving area efficient segmentation method based on depth feature compression convolutional network
A deep feature, driving area technology, applied in biological neural network models, image analysis, instruments, etc., can solve the problem that real-time is difficult to meet the requirements of intelligent vehicle environmental perception, to overcome accuracy and real-time requirements, reduce complexity degree, the effect of powerful feature expression ability
- Summary
- Abstract
- Description
- Claims
- Application Information
AI Technical Summary
Problems solved by technology
Method used
Image
Examples
Embodiment Construction
[0040] The technical solutions provided by the present invention will be described in detail below in conjunction with specific examples. It should be understood that the following specific embodiments are only used to illustrate the present invention and are not intended to limit the scope of the present invention.
[0041] The method for efficiently segmenting road driving areas based on deep feature compression convolutional networks provided by the present invention specifically includes the following steps:
[0042] (1) Establish a road segmentation data set, label the road samples obtained by the vehicle camera or use existing data samples, adjust the sample size to 227×227 pixels and record it as D k .
[0043](2) Design a deep feature compression convolutional neural network architecture, which consists of a fine feature extraction module and a layer-by-layer hierarchical decoupling module. In the feature extraction module, a standard convolutional layer is first desig...
PUM
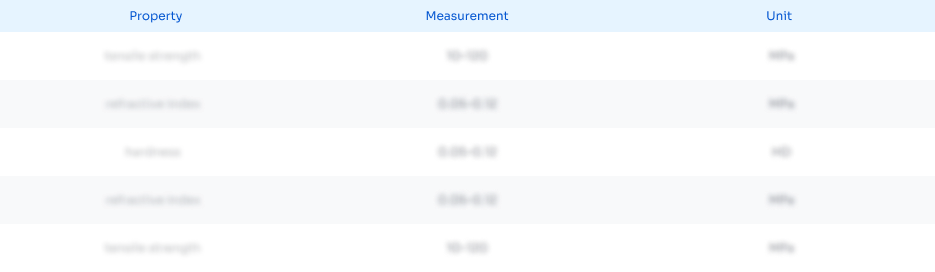
Abstract
Description
Claims
Application Information
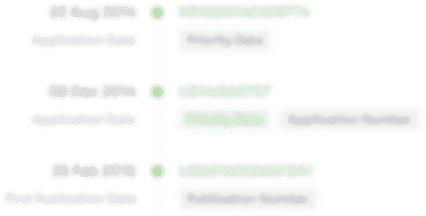
- R&D Engineer
- R&D Manager
- IP Professional
- Industry Leading Data Capabilities
- Powerful AI technology
- Patent DNA Extraction
Browse by: Latest US Patents, China's latest patents, Technical Efficacy Thesaurus, Application Domain, Technology Topic, Popular Technical Reports.
© 2024 PatSnap. All rights reserved.Legal|Privacy policy|Modern Slavery Act Transparency Statement|Sitemap|About US| Contact US: help@patsnap.com