LSTM hub single product energy consumption prediction based on incremental clustering
A technology of incremental clustering and hub, which is applied in the field of energy consumption prediction of LSTM hub single product based on incremental clustering, which can solve the problems of limited time modeling ability and learning long-term dependence
- Summary
- Abstract
- Description
- Claims
- Application Information
AI Technical Summary
Problems solved by technology
Method used
Image
Examples
Embodiment Construction
[0066] The present invention proposes a dynamic incremental density clustering algorithm based on PCA, that is, based on the characteristic parameter data symbolizing the production mode of the wheel hub, the clustering algorithm is used to obtain the historical item category similar to the new product; then, the Pearson coefficient and the Adaptive- The Lasso algorithm analyzes the strong explanatory factors of the energy consumption of a single product, and uses the BP neural network to predict the value of the strong explanatory factors of new products; finally, an ADE-based LSTM incremental update hub unit consumption prediction model is proposed, which uses ADE The algorithm weakens the impact of initialization parameters on model accuracy, and introduces an incremental learning strategy to realize dynamic update of the model.
[0067] One, the theoretical basis of the inventive method
[0068] 1. Principal Component Analysis (PCA): Transform the original data into a set ...
PUM
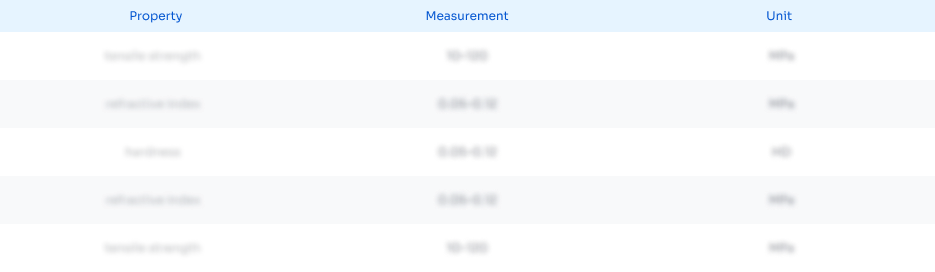
Abstract
Description
Claims
Application Information
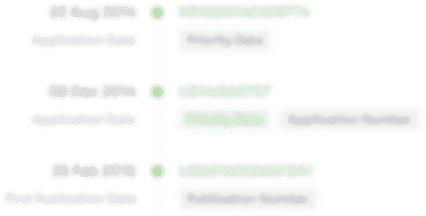
- R&D
- Intellectual Property
- Life Sciences
- Materials
- Tech Scout
- Unparalleled Data Quality
- Higher Quality Content
- 60% Fewer Hallucinations
Browse by: Latest US Patents, China's latest patents, Technical Efficacy Thesaurus, Application Domain, Technology Topic, Popular Technical Reports.
© 2025 PatSnap. All rights reserved.Legal|Privacy policy|Modern Slavery Act Transparency Statement|Sitemap|About US| Contact US: help@patsnap.com