Fault diagnosis method and system for rolling bearing based on relative entropy and k-nearest neighbor algorithm
A rolling bearing and fault diagnosis technology, which is applied in the direction of mechanical bearing testing, calculation, computer parts, etc., can solve the problems of bearing structure complexity, effect discounting, and increasing algorithm complexity, etc.
- Summary
- Abstract
- Description
- Claims
- Application Information
AI Technical Summary
Problems solved by technology
Method used
Image
Examples
Embodiment 2
[0128] A rolling bearing fault diagnosis system based on relative entropy and K nearest neighbor algorithm, such as figure 2 As shown, including data acquisition module 100, division module 200, relative entropy calculation module 300, model building module 400, data acquisition module 500 and diagnosis module 600 again;
[0129] The data acquisition module 100 is used to obtain the vibration data generated by the operation of the bearing in various fault states and the vibration data of the healthy state. The various fault states include at least the inner ring fault state, the outer ring fault state, and the rolling element fault status and cage failure status;
[0130] The division module 200 is used to perform equal-length partially overlapping sliding window interception and division on the collected vibration data to obtain division results;
[0131] The relative entropy calculation module 300 is used to calculate the relative relationship between the vibration data ge...
PUM
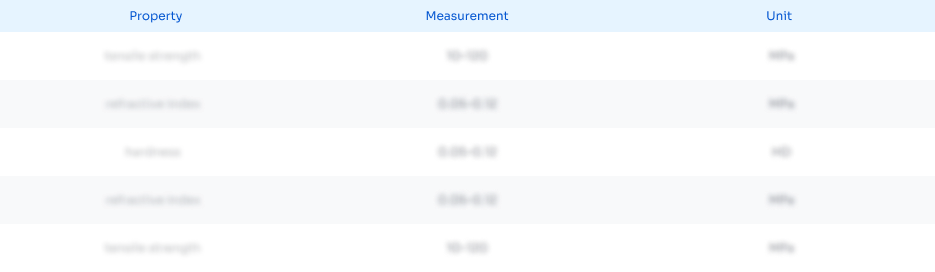
Abstract
Description
Claims
Application Information
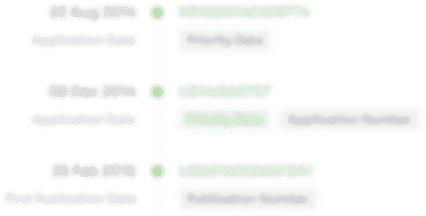
- R&D
- Intellectual Property
- Life Sciences
- Materials
- Tech Scout
- Unparalleled Data Quality
- Higher Quality Content
- 60% Fewer Hallucinations
Browse by: Latest US Patents, China's latest patents, Technical Efficacy Thesaurus, Application Domain, Technology Topic, Popular Technical Reports.
© 2025 PatSnap. All rights reserved.Legal|Privacy policy|Modern Slavery Act Transparency Statement|Sitemap|About US| Contact US: help@patsnap.com