Training method and device of semantic relation recognition model and terminal
A technology for semantic relationship and recognition model, applied in the training field of semantic relationship recognition model, can solve the problem of inaccurate semantic relationship recognition, and achieve the effect of improving classification effect, shortening prediction time, and improving prediction efficiency.
- Summary
- Abstract
- Description
- Claims
- Application Information
AI Technical Summary
Problems solved by technology
Method used
Image
Examples
Embodiment 1
[0065] In a specific embodiment, a training method of a semantic relationship recognition model is provided, such as figure 1 shown, including:
[0066] Step S10: input the sample data set into the initial pre-training model, output the representation information of the sample sentence, the sample data set includes a plurality of sample semantic units;
[0067] In an example, the sample data set includes a plurality of sample semantic units, and the sample semantic units may be basic semantic units serving as training data. The sample semantic unit may be a vocabulary or a word, for example, "taste", "good", "unpalatable" and so on. Multiple sample semantic units can form various sample semantic sentences, for example, "The taste is not bad, and the portion is also sufficient!", "But the environment is average, it is a comparison value for group buying, and friends can also go" and so on. A pre-trained model is a model trained on a large dataset. Pre-trained models can be m...
Embodiment 2
[0102] In a specific implementation, the multi-task learning process of sentiment analysis task and text relation task learning together is as follows: image 3 shown.
[0103] Split the chapter dataset into arg1 and arg2. arg1 and arg2 represent two EDU sentences and feed them into a BERT network learned on large-scale unsupervised data. In the input layer, arg1_1...arg1_i...arg1_n is the word vector representing the input arg1, and arg2_1...arg2_i...arg2_n is the word vector representing the input arg2. sep is a special character used for sentence segmentation, for example, space, comma, period, etc. cls (classification) is the characteristic character of the classification function.
[0104] arg1_1...arg1_i...arg1_n and arg2_1...arg2_i...arg2_n go through a multi-layer transformation network model (transformer model), and at the output layer, arg1_1...arg1_i...arg1_n is obtained as the representation word vector of output arg1, and arg2_1...arg2_i...arg2_n is the represe...
Embodiment 3
[0111] In another specific embodiment, a training device for a semantic relationship recognition model is provided, such as Figure 4 shown, including:
[0112] The representation information acquisition module 10 of the sample sentence is used to input the sample data set into the initial pre-training model, and output the representation information of the sample sentence, the sample data set includes a plurality of sample semantic units;
[0113] The feature word splicing module 20 is used to obtain a plurality of feature words, and splicing a plurality of feature words to obtain the representation information of the spliced feature words;
[0114] The semantic relationship category analysis module 30 is used to input the representation information of the sample sentence and the representation information of the spliced feature words into the initial classifier, and output the semantic relationship category between the sample semantic units;
[0115]Model adjustment mod...
PUM
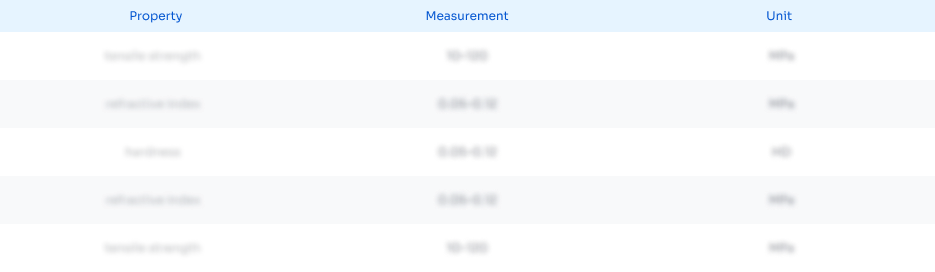
Abstract
Description
Claims
Application Information
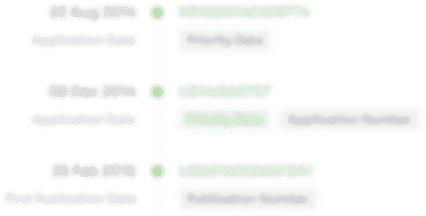
- R&D Engineer
- R&D Manager
- IP Professional
- Industry Leading Data Capabilities
- Powerful AI technology
- Patent DNA Extraction
Browse by: Latest US Patents, China's latest patents, Technical Efficacy Thesaurus, Application Domain, Technology Topic, Popular Technical Reports.
© 2024 PatSnap. All rights reserved.Legal|Privacy policy|Modern Slavery Act Transparency Statement|Sitemap|About US| Contact US: help@patsnap.com