Target tracking algorithm based on generative adversarial network
A target tracking and generative technology, applied in the field of image processing, can solve the problems of heavy wear and difficult operation.
- Summary
- Abstract
- Description
- Claims
- Application Information
AI Technical Summary
Problems solved by technology
Method used
Image
Examples
Embodiment 1
[0040] Such as Figure 1-4 Shown, a target tracking algorithm based on generative adversarial networks. When performing the target tracking algorithm, the generator in the generative confrontation network directly generates samples, and the discriminator generates a probability value to determine whether the sample is a probability value or a training value. In the process of moving target tracking, the particle filter framework is used to improve tracking. Finally, through offline training, the optimal parameter value of the generative confrontation network is obtained, and combined with the target tracking algorithm to realize the output of the graph.
[0041] The target tracking algorithm is specifically divided into the following steps:
[0042] S1: Determine the target in the video by manually framing the target;
[0043] S2: Extract the characteristic value of the target through the algorithm, and build the specific model of the target according to the characteristic v...
Embodiment 2
[0047] Such as Figure 1-4 As shown, according to the steps of Embodiment 1, the extraction of target features in S2 includes global and local feature extraction. On the basis of target features, a target model is constructed. The model is divided into a generative model G and a discriminative model D. The generative model is By calculating the joint probability of the target and the sample, find the sample closest to the target model as the estimate of the current target state, and the discriminant template is to calculate the conditional probability, directly judge whether the sample is the target, and realize the target through feature extraction and target model. Effectively distinguish from background features.
[0048] The specific objective function of the generated confrontation network is as follows:
[0049]
[0050] For any G, the optimal solution of D has the following form:
[0051]
[0052] Therefore, the objective function can be simplified as:
[0053] ...
Embodiment 3
[0056] Such as Figure 1-4 As shown, according to the steps of Embodiment 1 and 2, the offline network training in S3 is carried out in a semi-supervised manner, and the best training times are obtained by setting different training times, the training times of the discriminative model D and the training times of the generated model G during training. Generative adversarial network algorithm parameter values.
PUM
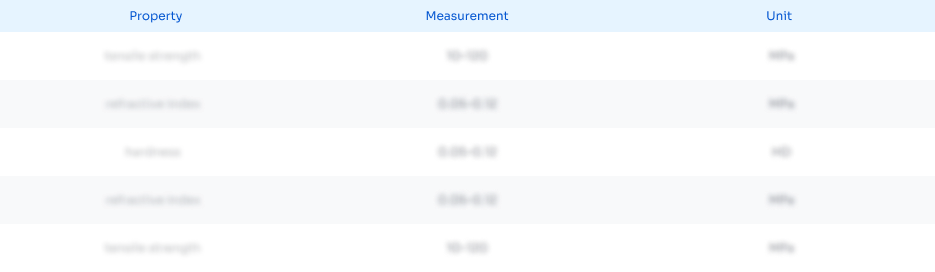
Abstract
Description
Claims
Application Information
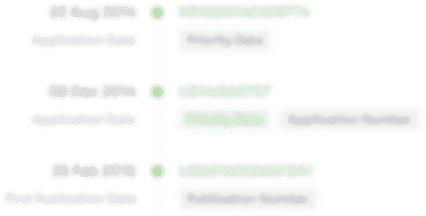
- R&D
- Intellectual Property
- Life Sciences
- Materials
- Tech Scout
- Unparalleled Data Quality
- Higher Quality Content
- 60% Fewer Hallucinations
Browse by: Latest US Patents, China's latest patents, Technical Efficacy Thesaurus, Application Domain, Technology Topic, Popular Technical Reports.
© 2025 PatSnap. All rights reserved.Legal|Privacy policy|Modern Slavery Act Transparency Statement|Sitemap|About US| Contact US: help@patsnap.com