Deep neural network target detection method integrated with pixel-level attention mechanism
A deep neural network and neural network technology, applied in the field of target detection in images, can solve problems such as inaccurate positioning, achieve strong generalization ability, simple implementation, and improve detection accuracy
- Summary
- Abstract
- Description
- Claims
- Application Information
AI Technical Summary
Benefits of technology
Problems solved by technology
Method used
Image
Examples
Embodiment Construction
[0026] The patent will be further described below in conjunction with the accompanying drawings and specific examples.
[0027] This patent can be applied to image object detection tasks, but is not limited to this task. Deep convolutional neural networks integrated with pixel-level attention mechanisms can also be used to solve many tasks that are sensitive to location, such as semantic segmentation. figure 1 is a flowchart of an example of the method. figure 2 A schematic structural diagram of a method for image target detection using a deep convolutional neural network integrated into a pixel-level attention mechanism of the present invention is described.
[0028] Applying the present invention to image target detection tasks mainly includes three steps: collecting images and preparing data sets; designing and training a deep convolutional neural network integrated into a pixel-level attention mechanism; testing / applying a detection model. The specific implementation st...
PUM
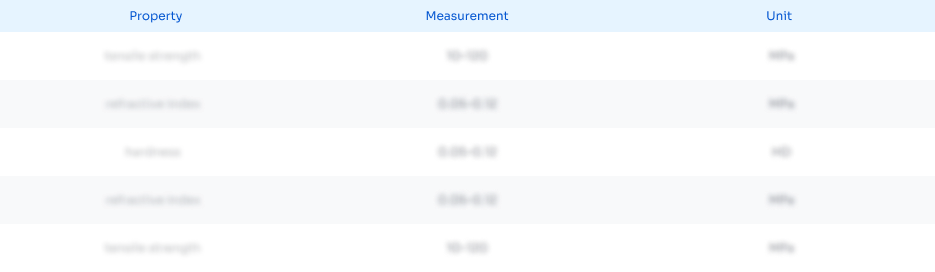
Abstract
Description
Claims
Application Information
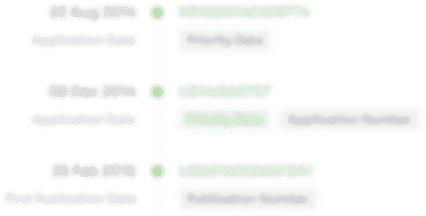
- R&D Engineer
- R&D Manager
- IP Professional
- Industry Leading Data Capabilities
- Powerful AI technology
- Patent DNA Extraction
Browse by: Latest US Patents, China's latest patents, Technical Efficacy Thesaurus, Application Domain, Technology Topic, Popular Technical Reports.
© 2024 PatSnap. All rights reserved.Legal|Privacy policy|Modern Slavery Act Transparency Statement|Sitemap|About US| Contact US: help@patsnap.com