Zero sample target classification method based on pseudo sample feature synthesis
A technology of object classification and pseudo-samples, applied in the field of zero-sample object classification based on the synthesis of pseudo-sample features, can solve the problems of low accuracy and achieve the effects of improving operating efficiency, ensuring richness, and avoiding domain drift problems
- Summary
- Abstract
- Description
- Claims
- Application Information
AI Technical Summary
Problems solved by technology
Method used
Image
Examples
Embodiment Construction
[0027] The present invention will be described in further detail below with reference to the accompanying drawings and specific embodiments.
[0028] like figure 1 Shown flow process, the concrete steps of the inventive method are as follows:
[0029] A zero-sample target classification method based on pseudo-sample feature synthesis, including the following steps:
[0030] Step 1: Name the category to be identified as the unseen category, first obtain the samples and annotation information of other categories similar to the category to be identified, and name it as the visible category dataset;
[0031] Step 2: Obtain the semantic information of all categories such as visible classes and unseen classes through web crawling.
[0032] Step 3: Calculate the similarity score between each unseen class and every other visible class category according to the semantic vector;
[0033] Step 4: Build a convolutional neural network classification model. The model is divided into two ...
PUM
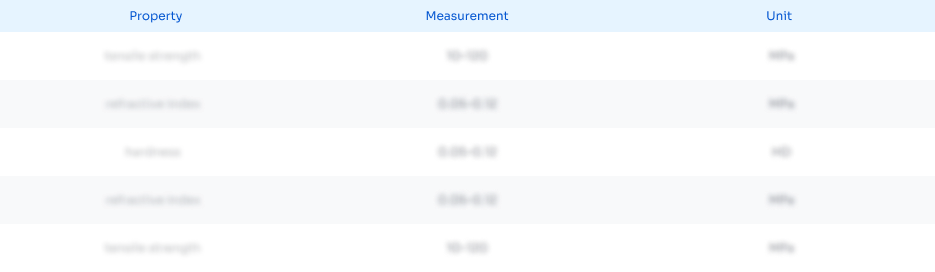
Abstract
Description
Claims
Application Information
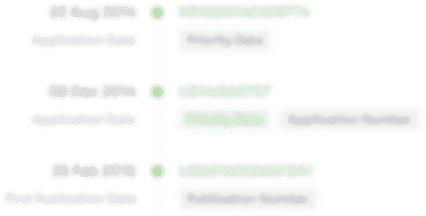
- R&D Engineer
- R&D Manager
- IP Professional
- Industry Leading Data Capabilities
- Powerful AI technology
- Patent DNA Extraction
Browse by: Latest US Patents, China's latest patents, Technical Efficacy Thesaurus, Application Domain, Technology Topic, Popular Technical Reports.
© 2024 PatSnap. All rights reserved.Legal|Privacy policy|Modern Slavery Act Transparency Statement|Sitemap|About US| Contact US: help@patsnap.com