Adversarial attack defense method based on adversarial sample training
A technology against samples and samples, applied in the field of artificial intelligence, can solve problems such as unreliable accuracy, and achieve the effect of improving robustness, suppressing overfitting, and enhancing generalization ability.
- Summary
- Abstract
- Description
- Claims
- Application Information
AI Technical Summary
Problems solved by technology
Method used
Image
Examples
Embodiment Construction
[0028] In order to facilitate those of ordinary skill in the art to understand and implement the present invention, the present invention will be described in further detail below in conjunction with the accompanying drawings and embodiments. It should be understood that the implementation examples described here are only used to illustrate and explain the present invention, and are not intended to limit this invention.
[0029] please see figure 1 , a method for adversarial attack defense based on adversarial sample training provided by the present invention, comprising the following steps:
[0030] Step 1: For the training set samples, introduce the prior distribution of the category labels of the training samples, and perform smooth correction on the labels; so that the network will not overly believe that the training samples are completely correct during training.
[0031] In order for the network not to fully trust the classification of the training samples, a uniform d...
PUM
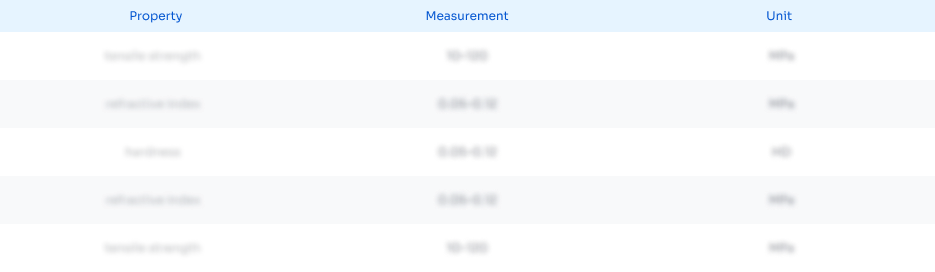
Abstract
Description
Claims
Application Information
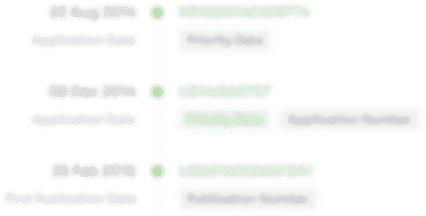
- R&D Engineer
- R&D Manager
- IP Professional
- Industry Leading Data Capabilities
- Powerful AI technology
- Patent DNA Extraction
Browse by: Latest US Patents, China's latest patents, Technical Efficacy Thesaurus, Application Domain, Technology Topic, Popular Technical Reports.
© 2024 PatSnap. All rights reserved.Legal|Privacy policy|Modern Slavery Act Transparency Statement|Sitemap|About US| Contact US: help@patsnap.com