Fire hazard early warning device based on smoke detection
A fire warning and smoke technology, applied in the field of image recognition, can solve the problems of reducing network model, reducing network parameters, etc., to achieve the effect of reducing network model, reducing network parameters, high classification accuracy and recognition speed
- Summary
- Abstract
- Description
- Claims
- Application Information
AI Technical Summary
Problems solved by technology
Method used
Image
Examples
no. 1 example
[0024] The video / image is first input to the convolutional layer 1 ( figure 1 Shown is a depthwise separable convolutional layer1). In the convolutional layer 1, convolution, batch normalization, and nonlinear function activation operations are sequentially performed, thereby obtaining the first convolutional data. Corresponding to an input image with a size of 64×64×3, the size of the first convolution data is 32×(64×64). Among them, batch normalization regularizes the data, while the nonlinear function uses the hyperbolic tangent (TanH) activation function.
[0025] Then, the first mean pooling layer performs first mean pooling processing on the first convolution data to obtain first mean pooling data with a size of 32×(32×32). The size of the first mean pooling layer is, for example, 3×3, and the stride is 2. Of course, those skilled in the art know that other sizes and step sizes can also be selected for mean pooling. In the present invention, the first mean pooling da...
no. 2 example
[0033] In a further preferred embodiment (the second embodiment), after the first pooling layer in the above-mentioned first embodiment and before the global mean pooling layer, a convolutional layer 3, a third mean pooling layer and a pooling layer can also be set 2 to further process the first feature map obtained in the merge layer 1 to enhance the stability, accuracy and robustness of the data.
[0034] As an example, in convolutional layer 3 ( figure 2 Shown is the depthwise separable convolutional layer 3), the first feature map is sequentially subjected to convolution, batch normalization and nonlinear function activation operations, and thus the third feature map with a size of 96×(32×32) is obtained. Convolve data. Among them, batch normalization regularizes the data, while the nonlinear function uses the ReLU activation function.
[0035] Then, the third mean pooling layer performs a third mean pooling process on the third convolutional data to obtain third mean p...
no. 3 example
[0040] In a further preferred embodiment (the third embodiment), after the merging layer 2 of the above-mentioned second embodiment and before the global mean pooling layer, a convolution layer 4, correcting the first mean pooling layer, and merging Layer 3, convolutional layer 5 and convolutional layer 6 further process the second feature map to further enhance the stability, accuracy and robustness of the data.
[0041] As an example, in convolutional layer 4 ( figure 2 Shown is the depthwise separable convolutional layer 4), the second feature map is sequentially subjected to convolution, batch normalization, nonlinear function activation, and maximum pooling layer operations, and thus obtains a size of 192×(16 ×16) of the fourth convolution data. Among them, the batch normalization regularizes the data, the nonlinear function uses the ReLU activation function, and the maximum pooling layer performs dimensionality reduction processing on the data. The size of the processi...
PUM
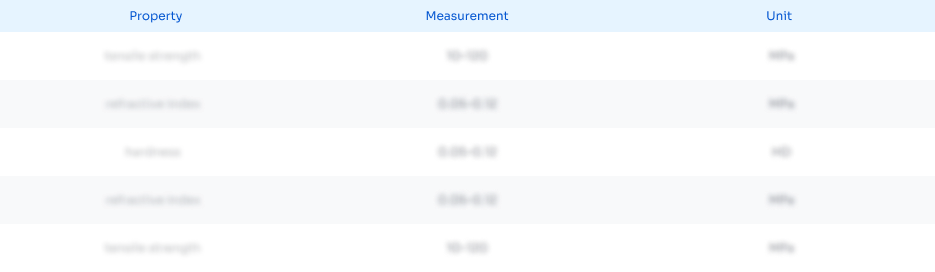
Abstract
Description
Claims
Application Information
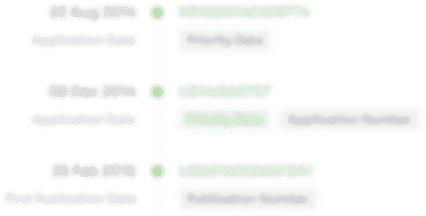
- R&D Engineer
- R&D Manager
- IP Professional
- Industry Leading Data Capabilities
- Powerful AI technology
- Patent DNA Extraction
Browse by: Latest US Patents, China's latest patents, Technical Efficacy Thesaurus, Application Domain, Technology Topic.
© 2024 PatSnap. All rights reserved.Legal|Privacy policy|Modern Slavery Act Transparency Statement|Sitemap